Text semantic similarity information processing method and system based on multi-model fusion
An information processing method and semantic similarity technology, applied in natural language data processing, semantic analysis, neural learning methods, etc., can solve the problems of unacceptable feedback time, large amount of calculation, waste of hardware resources, etc., to speed up real-time feedback speed , the effect of speeding up the feedback and reducing the amount of calculation
- Summary
- Abstract
- Description
- Claims
- Application Information
AI Technical Summary
Problems solved by technology
Method used
Image
Examples
Embodiment 1
[0108] For the patent data in the patent database, different models are used to process the title, abstract, claims, and instructions. Because there are large differences in the word frequency distribution, text length, and syntactic structure of each part, it is necessary to use different models for the four parts. deal with.
[0109] For titles and abstracts, because the length of the text is short, most of them are professional technical terms and their explanatory vocabulary, and the style is concise. Therefore, for the title abstract, word segmentation is first performed, and then keywords are extracted, and the keywords are sent to the word vector model. It is transformed into a corresponding word vector. The word vector model is used here because the word vector model is an unsupervised model that slides through the window in the article to intercept article fragments, such as Figure 4 As shown, using the intermediate vocabulary to predict the context vocabulary, the ...
Embodiment 2
[0132] Text semantic similarity information processing methods based on multi-model fusion include:
[0133] Step 1, perform word segmentation operations on the titles and abstracts of the papers in the paper database.
[0134] Step 2, use the title and abstract to train the word vector model.
[0135] Step 3: Split the full-text data in the paper into large chapters such as introduction, background, experiment, and effect comparison, and perform word segmentation for each chapter.
[0136] Step 4, use the word list of each chapter obtained in the above step 3 to train the sentence vector model.
[0137] Step 5, save the word vector model obtained in step 2 and the sentence vector model obtained in step 4.
[0138] Step 6, use the word vector model and the sentence vector model to perform sub-module feature extraction on the papers in the local paper database.
[0139] Step 7, build a feature fusion method, and fuse the features obtained in the above step 6.
[0140] Step ...
PUM
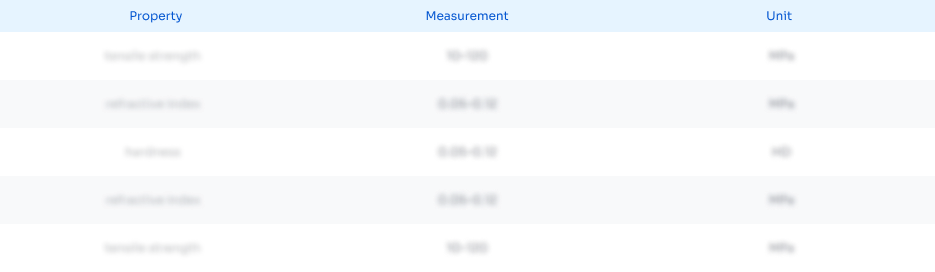
Abstract
Description
Claims
Application Information
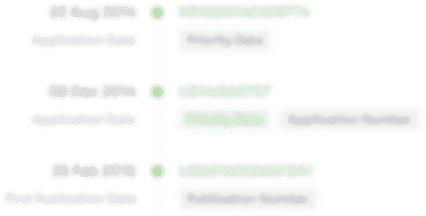
- R&D Engineer
- R&D Manager
- IP Professional
- Industry Leading Data Capabilities
- Powerful AI technology
- Patent DNA Extraction
Browse by: Latest US Patents, China's latest patents, Technical Efficacy Thesaurus, Application Domain, Technology Topic, Popular Technical Reports.
© 2024 PatSnap. All rights reserved.Legal|Privacy policy|Modern Slavery Act Transparency Statement|Sitemap|About US| Contact US: help@patsnap.com