Complex network node classification method based on graph attention network
A node classification, complex network technology, applied in neural learning methods, biological neural network models, comprehensive factory control, etc.
- Summary
- Abstract
- Description
- Claims
- Application Information
AI Technical Summary
Problems solved by technology
Method used
Image
Examples
Embodiment
[0086] The example selects 37 monitoring variables (the 38th variable is the introduced Gaussian noise) in the community that has a clear relationship with the steam turbine failure in Table 1 as the basis of the system network modeling. The data set used in this example comes from the monitoring data set of the enterprise DCS system, and the sampling frequency of the system is 1 / 60HZ. In this example, the samples collected from the steam turbine in the normal service state of the compressor unit for 5 consecutive months are selected to verify the method:
[0087] Step 1: Establishment of an undirected weighted network model based on coupled detrend correlation analysis
[0088] Calculate the coupling relationship between historical monitoring time series based on DCCA detrending correlation analysis, and introduce Gaussian noise series as a comparison of coupling correlation coefficients, and introduce a scaling index α as the lower threshold of the correlation coefficient. ...
PUM
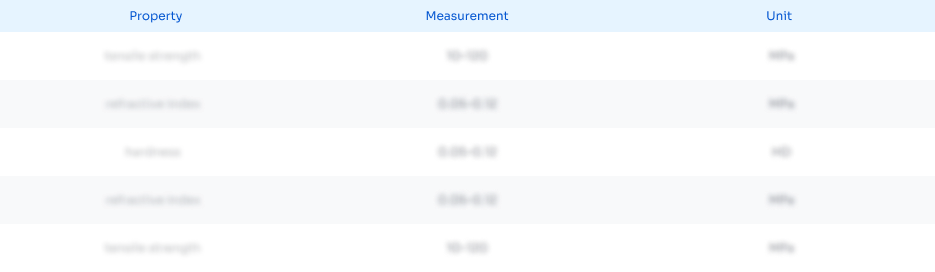
Abstract
Description
Claims
Application Information
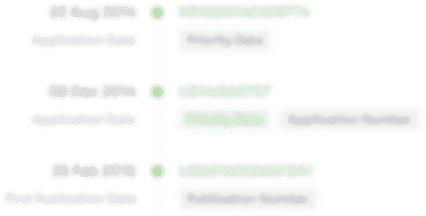
- R&D Engineer
- R&D Manager
- IP Professional
- Industry Leading Data Capabilities
- Powerful AI technology
- Patent DNA Extraction
Browse by: Latest US Patents, China's latest patents, Technical Efficacy Thesaurus, Application Domain, Technology Topic, Popular Technical Reports.
© 2024 PatSnap. All rights reserved.Legal|Privacy policy|Modern Slavery Act Transparency Statement|Sitemap|About US| Contact US: help@patsnap.com