Deep learning-based CBCT image cross-modal prediction CTA image stroke risk screening method and system
A deep learning and CT image technology, applied in the field of medical image processing, can solve problems such as irritation, leakage of intravenous contrast agent, skin damage, etc., to avoid repeated CT examinations and reduce radiation exposure dose.
- Summary
- Abstract
- Description
- Claims
- Application Information
AI Technical Summary
Problems solved by technology
Method used
Image
Examples
Embodiment
[0048] Such as figure 1 According to the stroke risk screening method for cross-modal prediction of CTA images based on deep learning CBCT images provided by the present invention, comprising the following steps:
[0049] Step 1: Build a cyclic adversarial generative network model;
[0050] Such as figure 2 As shown, it is a schematic structural diagram of the cyclic adversarial generation network model in the present invention, the cyclic adversarial generation network model includes: encoder, generator and discriminator, encoder, generator and discriminator mainly include: three-dimensional convolutional layer, ReLU layer (corrected linear unit layer), pooling layer, Batch Normalization layer (batch normalization layer) and Full Connect layer (full connection layer), the optimal step size of the convolutional layer: 3*3*3, the pooling layer uses Max pooling.
[0051] Step 101: use an encoder to encode high-order features;
[0052] The encoder is based on convolutional n...
PUM
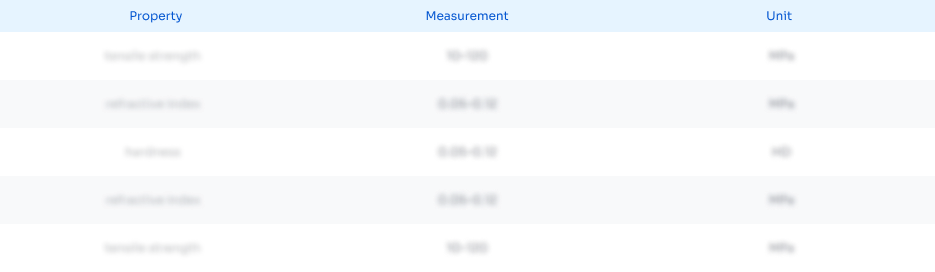
Abstract
Description
Claims
Application Information
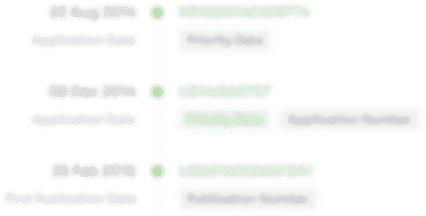
- R&D
- Intellectual Property
- Life Sciences
- Materials
- Tech Scout
- Unparalleled Data Quality
- Higher Quality Content
- 60% Fewer Hallucinations
Browse by: Latest US Patents, China's latest patents, Technical Efficacy Thesaurus, Application Domain, Technology Topic, Popular Technical Reports.
© 2025 PatSnap. All rights reserved.Legal|Privacy policy|Modern Slavery Act Transparency Statement|Sitemap|About US| Contact US: help@patsnap.com