Lightweight vehicle detection model construction method, system and device
A vehicle detection and lightweight technology, which is applied in the traffic control system of road vehicles, traffic control system, character and pattern recognition, etc., can solve the problems of slow detection speed and low detection accuracy, and achieve the goal of improving detection accuracy and detection speed Effect
- Summary
- Abstract
- Description
- Claims
- Application Information
AI Technical Summary
Problems solved by technology
Method used
Image
Examples
Embodiment 1
[0102] An embodiment of the present invention provides a device for constructing a lightweight vehicle detection model based on deep learning, such as Figure 10 As shown, it includes: a memory 100, a processing 102, and a computer program stored on the memory 100 and operable on the processor 102. When the computer program is executed by the processor 102, the following method steps are implemented:
[0103] Step 101, add a lightweight channel attention module at the end of the bidirectional feature pyramid module of the EfficientNet target detection algorithm EfficientDet model as the backbone network, and learn different channels through the channel attention module with a one-dimensional convolution with a certain step size The relationship between the feature maps; step 101 specifically includes:
[0104] Through the channel attention module, the input C-dimensional feature map is converted into a 1*1*C vector by using global average pooling, and then a one-dimensional co...
Embodiment 2
[0131] An embodiment of the present invention provides a computer-readable storage medium, where a program for realizing information transmission is stored on the computer-readable storage medium, and when the program is executed by the processor 102, the following method steps are implemented:
[0132] Step 101, add a lightweight channel attention module at the end of the bidirectional feature pyramid module of the EfficientNet target detection algorithm EfficientDet model as the backbone network, and learn different channels through the channel attention module with a one-dimensional convolution with a certain step size The relationship between the feature maps; step 101 specifically includes:
[0133] Through the channel attention module, the input C-dimensional feature map is converted into a 1*1*C vector by using global average pooling, and then one-dimensional convolution with a convolution kernel size of k is performed, and then learned through the Sigmoid function outpu...
PUM
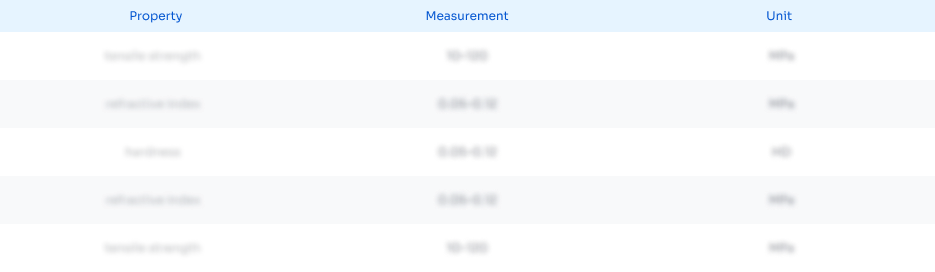
Abstract
Description
Claims
Application Information
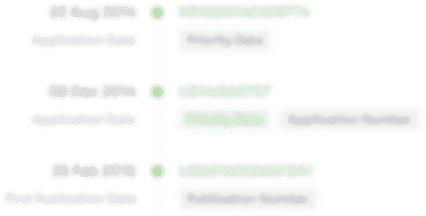
- R&D Engineer
- R&D Manager
- IP Professional
- Industry Leading Data Capabilities
- Powerful AI technology
- Patent DNA Extraction
Browse by: Latest US Patents, China's latest patents, Technical Efficacy Thesaurus, Application Domain, Technology Topic, Popular Technical Reports.
© 2024 PatSnap. All rights reserved.Legal|Privacy policy|Modern Slavery Act Transparency Statement|Sitemap|About US| Contact US: help@patsnap.com