Enhanced multi-scale convolutional neural network soft measurement method
A convolutional neural network and soft-sensing technology, applied in the field of industrial process soft-sensing, can solve the problems of not getting soft-sensing effect, bad model, influence, etc., and achieve the effect of expanding the absolute quantity
- Summary
- Abstract
- Description
- Claims
- Application Information
AI Technical Summary
Problems solved by technology
Method used
Image
Examples
Embodiment Construction
[0029] The enhanced multi-scale convolution kernel CNN soft-sensing method of the present invention will be further described in detail below in combination with specific implementation methods.
[0030] An enhanced multi-scale convolutional neural network soft-sensing method of the present invention is based on a generative confrontation network and a convolutional neural network (CNN) structure to realize the prediction of quality variable data in industry, including the following steps:
[0031] Step 1 (data preprocessing): the data preprocessing process is as follows figure 1 As shown, let the quality variable be Y and the process variable be X i , i=1,2,...,m, m is the number of process variables. Take the quality variable data collected at time t as the sample S t output value y t , t=1,2,.... Assuming that the duration of the material in the entire process is n, the process variable x collected during the [t-n, t] time period i·j , i=1,2,...,m, j=1,2,...,n, spliced...
PUM
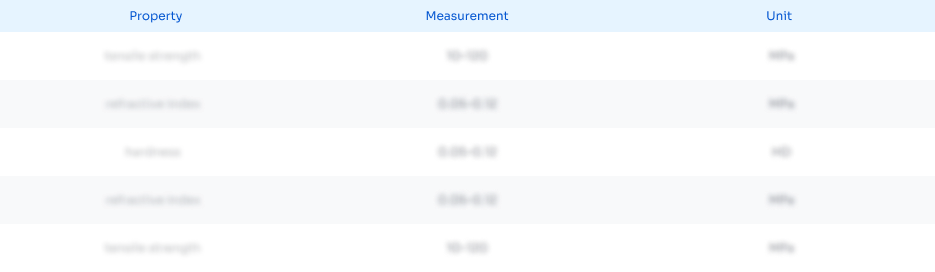
Abstract
Description
Claims
Application Information
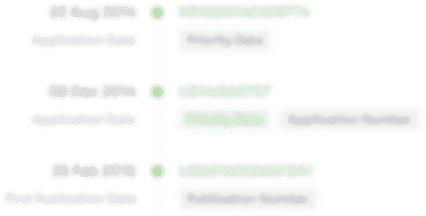
- R&D Engineer
- R&D Manager
- IP Professional
- Industry Leading Data Capabilities
- Powerful AI technology
- Patent DNA Extraction
Browse by: Latest US Patents, China's latest patents, Technical Efficacy Thesaurus, Application Domain, Technology Topic, Popular Technical Reports.
© 2024 PatSnap. All rights reserved.Legal|Privacy policy|Modern Slavery Act Transparency Statement|Sitemap|About US| Contact US: help@patsnap.com