Feature fusion method of multi-modal deep neural network
A feature fusion and multi-modal technology, applied in the field of medical imaging and deep learning, can solve the problems of feature weight distribution analysis and processing, and achieve the effect of maximizing performance
- Summary
- Abstract
- Description
- Claims
- Application Information
AI Technical Summary
Problems solved by technology
Method used
Image
Examples
Embodiment Construction
[0016] Taking the PET / CT dual mode as an example (that is, when x=2), the present invention will be described in detail in conjunction with the accompanying drawings.
[0017] Such as figure 1 Shown, the inventive method specifically comprises the following steps:
[0018] Step 1: In the dual-branch dual-modal 3D CNN, the two branches correspond to the convolution branch of the PET modality and the convolution branch of the CT modality respectively. For the 3D feature maps output by the nth level of the two 3D convolution branches, the 3D feature maps of the two modalities are superimposed on the channel dimension to obtain a 3D feature map with twice the number of original channels. Then perform average pooling in the three dimensions of depth, height, and width, and compress the three dimensions of depth, height, and width to obtain a one-dimensional vector of a channel dimension. After downsampling and upsampling with a compression ratio of 16:1:16, and using the activati...
PUM
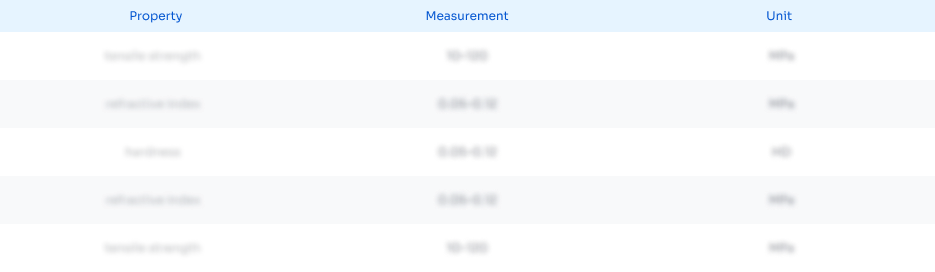
Abstract
Description
Claims
Application Information
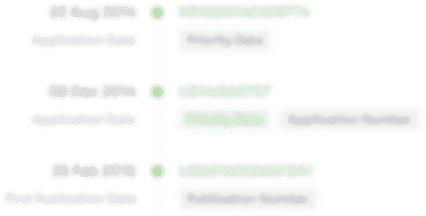
- R&D Engineer
- R&D Manager
- IP Professional
- Industry Leading Data Capabilities
- Powerful AI technology
- Patent DNA Extraction
Browse by: Latest US Patents, China's latest patents, Technical Efficacy Thesaurus, Application Domain, Technology Topic, Popular Technical Reports.
© 2024 PatSnap. All rights reserved.Legal|Privacy policy|Modern Slavery Act Transparency Statement|Sitemap|About US| Contact US: help@patsnap.com