Scene semantic segmentation method based on deep learning
A semantic segmentation and deep learning technology, applied in neural learning methods, instruments, biological neural network models, etc., can solve the problem that the target contour cannot be divided and recognized in detail, and achieve the effect of ensuring stability
- Summary
- Abstract
- Description
- Claims
- Application Information
AI Technical Summary
Problems solved by technology
Method used
Image
Examples
Embodiment Construction
[0060] The core idea of the present invention is to provide a scene semantic segmentation method based on deep learning, which can effectively distinguish the recognition accuracy of the scene outline, thereby improving MIoU. In order to make the purpose, technical solutions and advantages of the present invention clearer, the present invention will be further described in detail in conjunction with the accompanying drawings and embodiments. The specific embodiments described below are only used to explain the present invention and are not intended to limit the present invention. The main idea of the invention.
[0061] The embodiment of the present invention and its implementation process are as follows. The overall implementation block diagram of its convolutional neural network is shown in Figure 1, which includes two processes, the training phase and the testing phase;
[0062] Step 1: Select the semantic segmentation training data set; in this embodiment, PASCAL VOC 2...
PUM
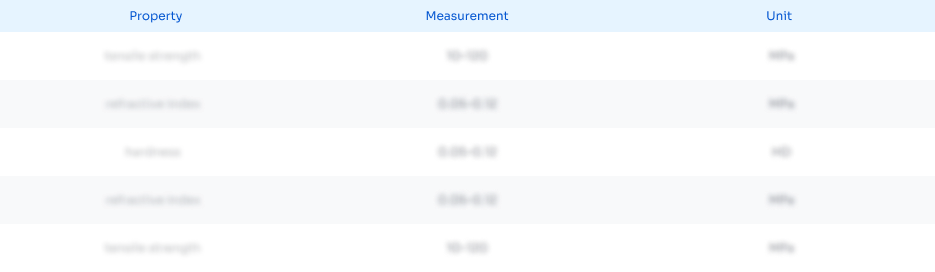
Abstract
Description
Claims
Application Information
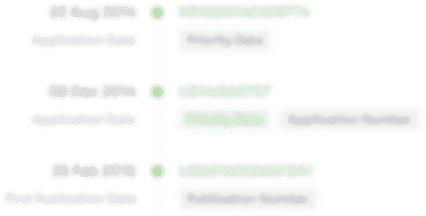
- R&D
- Intellectual Property
- Life Sciences
- Materials
- Tech Scout
- Unparalleled Data Quality
- Higher Quality Content
- 60% Fewer Hallucinations
Browse by: Latest US Patents, China's latest patents, Technical Efficacy Thesaurus, Application Domain, Technology Topic, Popular Technical Reports.
© 2025 PatSnap. All rights reserved.Legal|Privacy policy|Modern Slavery Act Transparency Statement|Sitemap|About US| Contact US: help@patsnap.com