Text analysis method based on various deep topic models
A topic model, text analysis technology, applied in text database clustering/classification, unstructured text data retrieval, special data processing applications, etc. Training and other issues to achieve the effect of improving text analysis capabilities, improving model practicability, and fast parallel training
- Summary
- Abstract
- Description
- Claims
- Application Information
AI Technical Summary
Problems solved by technology
Method used
Image
Examples
Embodiment Construction
[0032] The present invention will be described in further detail below in conjunction with specific examples, but the embodiments of the present invention are not limited thereto.
[0033] See figure 1 , figure 1 It is a block diagram of steps of a text analysis method based on a variety of deep topic models provided by an embodiment of the present invention, including:
[0034] Construct a training sample set and a test sample set of text data;
[0035] Construct a variety of deep topic models according to the training sample set, and initialize the initial model parameters of the various deep topic models;
[0036] Training a variety of deep topic models according to the training sample set to obtain training model parameters, and updating the initial model parameters according to the training model parameters to obtain a variety of deep topic models after training;
[0037] According to the test sample set, train the various deep topic models after training to obtain som...
PUM
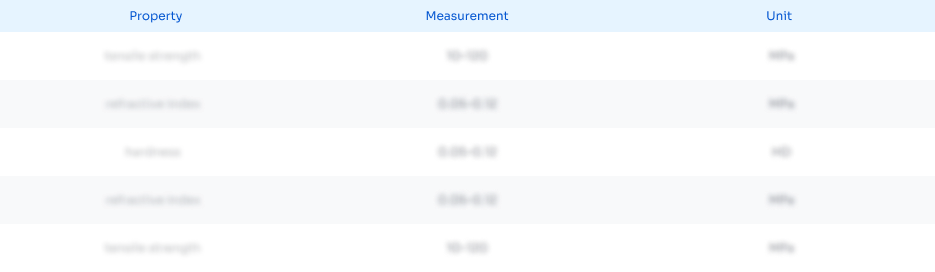
Abstract
Description
Claims
Application Information
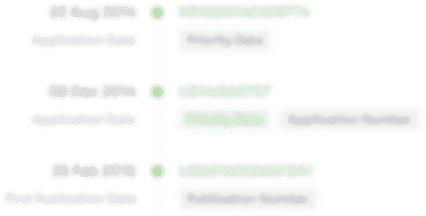
- R&D
- Intellectual Property
- Life Sciences
- Materials
- Tech Scout
- Unparalleled Data Quality
- Higher Quality Content
- 60% Fewer Hallucinations
Browse by: Latest US Patents, China's latest patents, Technical Efficacy Thesaurus, Application Domain, Technology Topic, Popular Technical Reports.
© 2025 PatSnap. All rights reserved.Legal|Privacy policy|Modern Slavery Act Transparency Statement|Sitemap|About US| Contact US: help@patsnap.com