Reasoning method and system for dynamic branch selection of deep learning model
A technology of deep learning and reasoning method, applied in the field of artificial intelligence deep learning and reasoning, it can solve the problems of feature redundancy and insufficient reasoning speed of edge computing equipment, and achieve the effect of improving reasoning speed and reducing computational redundancy.
- Summary
- Abstract
- Description
- Claims
- Application Information
AI Technical Summary
Problems solved by technology
Method used
Image
Examples
Embodiment Construction
[0019] Such as figure 1 as well as figure 2 As shown, the embodiment of this specification provides a reasoning method for dynamic branch selection of a deep learning model, including:
[0020] S101. Construct and train a deep learning model for semantic classification, semantic detection or semantic segmentation of pictures. The deep learning model includes an encoder 11, a branch selector 12, and a plurality of decoding branches 13 corresponding to different branch categories one by one, The deep learning model is trained as:
[0021] A plurality of primary features (intermediate features) are extracted from the input picture by the encoder 11 .
[0022] The branch classes of the primary features are identified and output by the branch selector 12 .
[0023] The decoding branch 13 processes all the primary features through the feature weight group corresponding to the branch category to obtain deep features, recognizes the deep features and outputs the inference result. ...
PUM
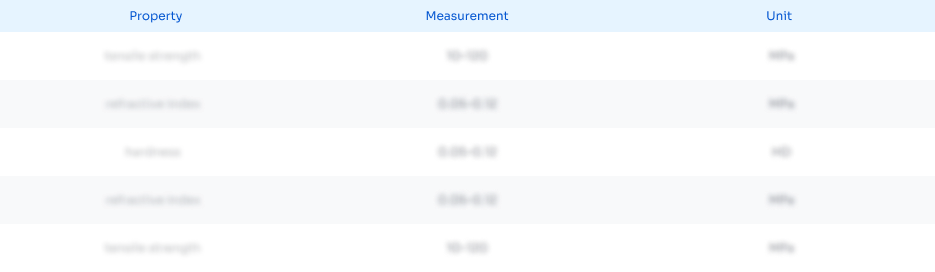
Abstract
Description
Claims
Application Information
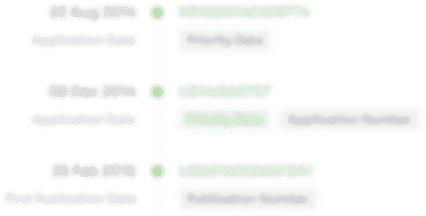
- R&D Engineer
- R&D Manager
- IP Professional
- Industry Leading Data Capabilities
- Powerful AI technology
- Patent DNA Extraction
Browse by: Latest US Patents, China's latest patents, Technical Efficacy Thesaurus, Application Domain, Technology Topic, Popular Technical Reports.
© 2024 PatSnap. All rights reserved.Legal|Privacy policy|Modern Slavery Act Transparency Statement|Sitemap|About US| Contact US: help@patsnap.com