Data selection method in federated learning scene
A data selection and scenario technology, applied in machine learning, digital data protection, electronic digital data processing, etc., can solve the problems that data cannot be directly accessed by third parties, reduce model performance, and not be considered, so as to achieve efficient and efficient selection strategies. Efficient and accurate results of data selection strategies
- Summary
- Abstract
- Description
- Claims
- Application Information
AI Technical Summary
Problems solved by technology
Method used
Image
Examples
Embodiment Construction
[0018] The present invention will be described in detail below in conjunction with accompanying drawing: figure 1 As shown, the data selection method in the federated learning scenario proposed by the present invention is mainly divided into the following modules: filtering out users and data related to tasks, user selection before training, user and data selection during training, and model training.
[0019] (1) Task-related user and data filtering: when a FL task arrives, the server first calculates each user C k , the label set Y of k ∈ [K] k ={y k |(x k ,y k )∈D k} and the intersection of the target label set Y {(x k ,y k )|y k ∈ Y k ∩Y} to filter out users with data of the target category. If the number of samples in the intersection set exceeds the minimum number of target models|{(x k ,y k )|y k ∈ Y k ∩Y}|>v, the user is relevant. In order to meet the needs of privacy protection, we use privacy protection intersection technology (PSI).
[0020] (2) User ...
PUM
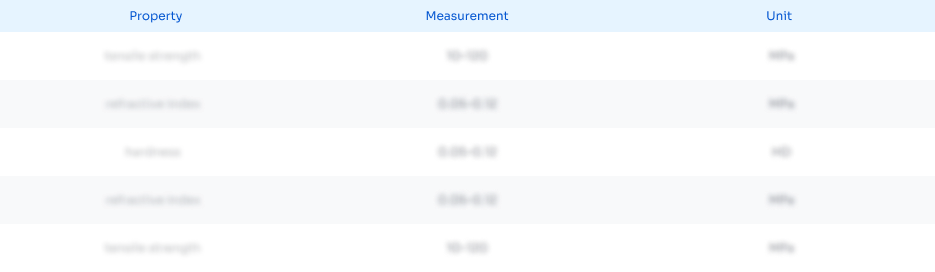
Abstract
Description
Claims
Application Information
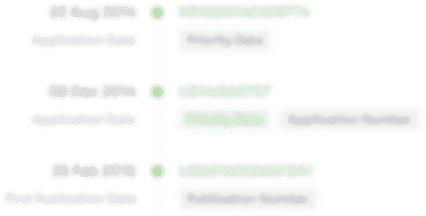
- R&D
- Intellectual Property
- Life Sciences
- Materials
- Tech Scout
- Unparalleled Data Quality
- Higher Quality Content
- 60% Fewer Hallucinations
Browse by: Latest US Patents, China's latest patents, Technical Efficacy Thesaurus, Application Domain, Technology Topic, Popular Technical Reports.
© 2025 PatSnap. All rights reserved.Legal|Privacy policy|Modern Slavery Act Transparency Statement|Sitemap|About US| Contact US: help@patsnap.com