Quantum fuzzy machine learning adversarial defense model method
A machine learning and quantum technology, applied in quantum computers, computing models, integrated learning, etc., to achieve high robustness, improved robustness, and wide defense applications
- Summary
- Abstract
- Description
- Claims
- Application Information
AI Technical Summary
Problems solved by technology
Method used
Image
Examples
Embodiment 1
[0045] A quantum fuzzy machine learning confrontation defense model method, its flow chart is as follows figure 1 As shown, the details are as follows:
[0046] S1. Constructing a quantum fuzzy data sample of a legitimate user;
[0047] First, according to the quantum fuzzy mathematical management model, fuzzy sets are introduced, and the classic fuzzy data sample set is: D={i ,y i ,μ i (x i )>|x i ∈X},y i ∈{-1,+1},
[0048] Among them, μ i (x i ) for x i Belongs to the fuzzy set {i ,μ A (x i )>|x i ∈X} membership function, each x i =(x i1 ,x i2 ,...,x im ) m eigenvectors are coded into the quantum probability amplitude to form the quantum probability amplitude code, the process can be expressed as:
[0049] Represents a normalized vector;
[0050] Secondly, the quantum fuzzy data sample is prepared, and the quantum fuzzy data sample is expressed as:
[0051] in, x i is the i-th data sample, y i There are only two values (+1 or -1).
[0052] S2. ...
Embodiment 2
[0062] Based on the above embodiment 1, its flow chart is as follows figure 1 and 2 As shown, when the adversarial defense module adopts the first type of defense strategy; that is, the quantum fuzzy machine learning adversarial sample recognizer, it can prevent the samples submitted by malicious attackers and achieve the purpose of defense.
[0063] Among them, the defense method of quantum fuzzy machine learning against sample identifier is as follows:
[0064] The quantum fuzzy machine learning adversarial example recognizer uses a binary classification method for training and testing, and identifies quantum fuzzy data samples submitted by legitimate users to make correct decisions; identify quantum fuzzy adversarial examples submitted by malicious attackers Block the attack of this sample.
[0065] Among them, the two classification methods include:
[0066] Divide the quantum fuzzy data samples into a test set of quantum fuzzy data samples and the training set of ...
Embodiment 3
[0070] Based on the above embodiment 1, its flow chart is as follows figure 1 and 3 As shown, when the adversarial defense module adopts the second type of defense strategy, the second type of defense strategy is to reconstruct the input quantum fuzzy mixed samples (including quantum fuzzy data samples and quantum fuzzy confrontation samples), so that the quantum fuzzy machine learning system can do Make the right decision to achieve the goal of defense.
[0071] Quantum fuzzy machine learning defenses against sample correctors include:
[0072] Input the quantum fuzzy mixed sample, the quantum fuzzy mixed sample is the sum of the legitimate user's quantum fuzzy data sample and the malicious attacker's quantum fuzzy confrontation sample;
[0073] The quantum fuzzy mixed samples are corrected by the reconstruction method, so that the quantum fuzzy machine learning system can make correct decisions.
[0074] Among them, the reconstruction method is to use the self-encoding me...
PUM
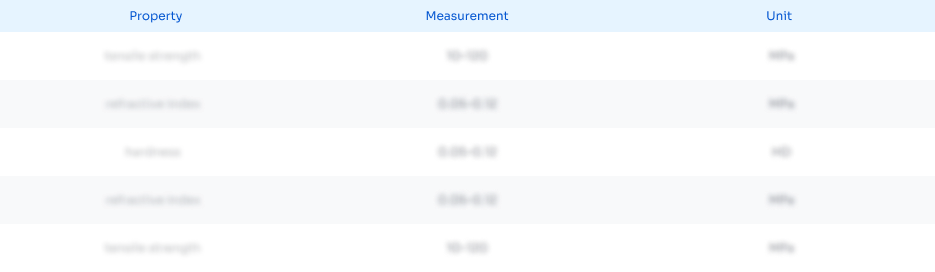
Abstract
Description
Claims
Application Information
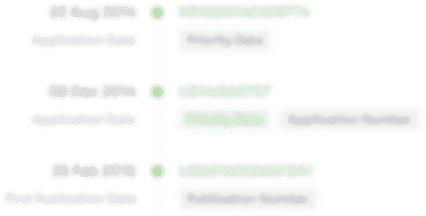
- Generate Ideas
- Intellectual Property
- Life Sciences
- Materials
- Tech Scout
- Unparalleled Data Quality
- Higher Quality Content
- 60% Fewer Hallucinations
Browse by: Latest US Patents, China's latest patents, Technical Efficacy Thesaurus, Application Domain, Technology Topic, Popular Technical Reports.
© 2025 PatSnap. All rights reserved.Legal|Privacy policy|Modern Slavery Act Transparency Statement|Sitemap|About US| Contact US: help@patsnap.com