Health data detection method based on convolution auto-encoder Gaussian mixture model
A Gaussian mixture model, convolutional self-encoding technology, applied in the field of healthcare, can solve problems such as high computational overhead, achieve the effect of improving accuracy and avoiding computational overhead
- Summary
- Abstract
- Description
- Claims
- Application Information
AI Technical Summary
Problems solved by technology
Method used
Image
Examples
Embodiment 1
[0059] This embodiment provides a health data detection method based on a convolutionary self-encoder Gaussian mixed model, the method comprising:
[0060] Step 1: Training the collected original high-dimensional health data is trained from the encoder to minimize the reconstruction error, combined with the BP optimization algorithm to obtain the low-dimensional representation of the original high-dimensional health data and corresponding Reconstruction error;
[0061] Step 2: The sample density corresponding to the original high-dimensional health data is calculated as the original high-dimensional health data of the original high-dimensional health data, and the maximum value of the sample density is not only the density threshold, and the EM algorithm is combined to the Gaussian mixture model. Training, to obtain the optimal Gaussian mixed model parameters;
[0062] During the calculation, the CHOLESKY decomposition of the covariance matrix is constructed by the mixing probab...
Embodiment 2
[0066] This embodiment provides a health data detection method based on convolutionautoencododer gaussian mixture model (CAE-GMM), which first uses MIN-Max normalization to normalize data samples. Due to the "dimension disaster", the density estimate is very difficult by conventional methods, so data samples are trained on the convolution from the encoder until the reconstruction error reaches the minimum, and this nonlinear manner is reduced in reducing "dimension disaster" While accurately acquiring the potential spatial representation of data samples as much as possible;
PUM
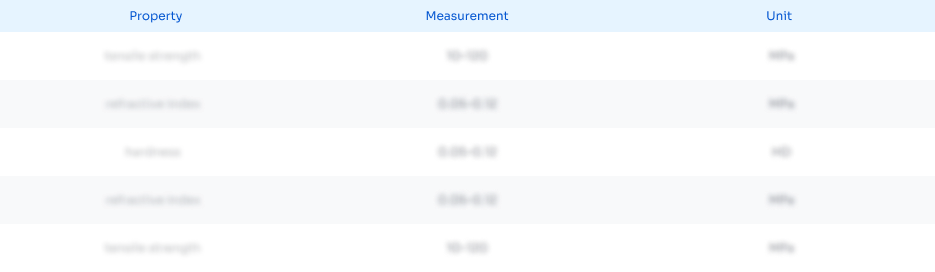
Abstract
Description
Claims
Application Information
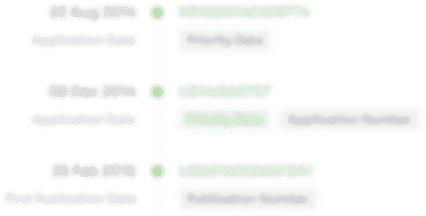
- R&D Engineer
- R&D Manager
- IP Professional
- Industry Leading Data Capabilities
- Powerful AI technology
- Patent DNA Extraction
Browse by: Latest US Patents, China's latest patents, Technical Efficacy Thesaurus, Application Domain, Technology Topic, Popular Technical Reports.
© 2024 PatSnap. All rights reserved.Legal|Privacy policy|Modern Slavery Act Transparency Statement|Sitemap|About US| Contact US: help@patsnap.com