Method and system for improving video question-answering precision based on multi-modal fusion model
A fusion model and multi-modal technology, applied in the field of natural language processing and deep learning, can solve problems such as large answer error, extraction, and difficulty in meeting the accuracy requirements of video question and answer, and achieve the goal of improving test accuracy and accuracy Effect
- Summary
- Abstract
- Description
- Claims
- Application Information
AI Technical Summary
Problems solved by technology
Method used
Image
Examples
Embodiment 1
[0045] Such as figure 1 As shown, the present disclosure provides a method for improving video question answering accuracy based on a multimodal fusion model, including:
[0046] Collect video data and question features, and obtain video question-and-answer questions;
[0047] Extract visual features and subtitle features from video data;
[0048] Perform fusion processing of visual features and subtitle features to obtain fused visual features and fused subtitle features;
[0049] Input the fused visual features, fused subtitle features and question features into the multimodal fusion model for training to obtain a trained multimodal fusion model;
[0050] Input the questions of video question answering into the trained multimodal fusion model, obtain the answers to the questions, and predict the probability of each answer being the correct answer.
[0051] Further, the collection of video data and question features, and the acquisition of video question-and-answer questio...
Embodiment 2
[0091] Such as figure 2 As shown, the framework of this disclosure aims to select the correct answer in video question answering.
[0092] The TVQA dataset is a benchmark for video question answering, containing 152,545 human-annotated multiple-choice question-answer pairs (84,768 what, 13,644 how, 17,777 where, 15,798 why, 17,654 who asked), from 6 TV shows (“ 21.8K video clips of The Big Bang Theory, Castle, How I Met Ben's Mother, Grey's Anatomy, MD's House, Friends). Questions in the TVQA dataset have five candidate answers, only one of which is the correct answer. The format of the test questions in the dataset is designed as follows:
[0093]"[What / How / Where / Why / who]___[when / before / after / …]___", both parts of the question require visual and verbal understanding. There are a total of 122,039 QAs in the training set, 15,253 QAs in the validation set, and 7,623 QAs in the test set.
[0094] Evaluations for this disclosure were performed on a computer equipped with an I...
Embodiment 3
[0101] A system for improving the accuracy of video question answering based on a multimodal fusion model, including:
[0102] The data collection module is configured to: collect video data and question features, and obtain video question-and-answer questions;
[0103] The data processing module is configured to: extract visual features and subtitle features from video data;
[0104] The feature fusion module is configured to: perform fusion processing on visual features and subtitle features to obtain fusion visual features and fusion subtitle features;
[0105] The model training module is configured to: input the fusion visual feature, the fusion subtitle feature and the question feature into the multimodal fusion model for training, and obtain the trained multimodal fusion model;
[0106] The output module is configured to: input the question of the video question answering into the trained multi-modal fusion model, and use the multi-head self-attention mechanism to obta...
PUM
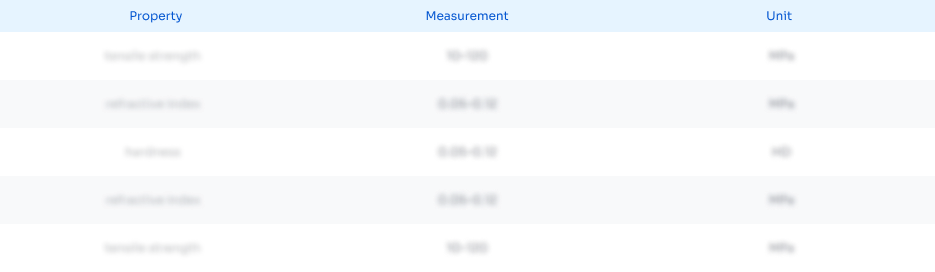
Abstract
Description
Claims
Application Information
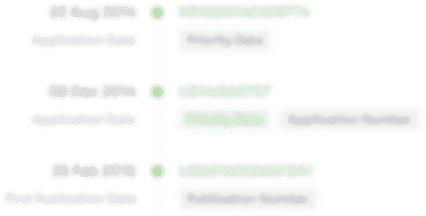
- R&D Engineer
- R&D Manager
- IP Professional
- Industry Leading Data Capabilities
- Powerful AI technology
- Patent DNA Extraction
Browse by: Latest US Patents, China's latest patents, Technical Efficacy Thesaurus, Application Domain, Technology Topic, Popular Technical Reports.
© 2024 PatSnap. All rights reserved.Legal|Privacy policy|Modern Slavery Act Transparency Statement|Sitemap|About US| Contact US: help@patsnap.com