Control method for adjusting multi-screen brightness through face tracking based on artificial intelligence
A technology of artificial intelligence and control method, applied in neural learning methods, computer parts, cathode ray tube indicators, etc., can solve problems such as dry facial skin, eye diseases, and corneal dryness.
- Summary
- Abstract
- Description
- Claims
- Application Information
AI Technical Summary
Problems solved by technology
Method used
Image
Examples
no. 1 example
[0024] The first embodiment, such as figure 1 and image 3 .
[0025] The training process of the neural network can be divided into the following steps.
[0026] Step 1: Define the neural network, including some learnable parameters or weights.
[0027] Step 2: Input the data into the network for training and calculate the loss value.
[0028] Step 3: Backpropagate the gradient to the parameters or weights of the network, update the weights of the network accordingly, and train again.
[0029] Step 4: Save the final trained model.
[0030] After the face image is input into the neural network, features are extracted through convolution operations, and then output to the next layer after down-sampling operations. After multiple convolution, activation, and pooling layers, the result is output to the fully connected layer, and then mapped to the final classification result after fully connected: nine face orientations.
[0031] Load the model for facial orientation recogn...
no. 2 example
[0034] Because the original image acquired by the camera device will be subject to various conditions and random interference, it is often not used directly, and it must be pre-processed in the early stage of image processing, such as grayscale correction and noise filtering. The preprocessing process mainly includes light compensation, grayscale transformation, histogram equalization, normalization, filtering and sharpening of face images. To put it simply, it is to finely process the captured images, and divide the detected faces into pictures of a certain size for easy recognition and processing.
[0035] In the neural network, first input an image, the size of the input image is W × H × 3, then convolve the input image with the convolution kernel, and then perform activation and pooling operations. The convolution process uses 6 convolution kernels, Each convolution kernel has three channels of R, G, and B. The number of channels of the convolution kernel is the same as th...
no. 3 example
[0044] The third embodiment, such as Figure 1-Figure 3 .
[0045] The present invention includes the following steps.
[0046] Step S11: Enter the parameter collection stage before neural network training and learning: Divide the face directions into nine types, namely up, down, left, right, upper left, upper right, lower left, lower right and middle directions, and collect these nine facial directions images are used as training set samples. The image information of various facial orientations is used as input parameters before neural network training and learning, and nine facial orientations are used as output parameters before neural network training and learning.
[0047] Step S12: Establish a face orientation-display ID comparison table.
[0048] Step S13: Entering the neural network training and learning stage: the neural network is trained and learned according to known input parameters and output parameters, and the learning results are used as hidden layer data i...
PUM
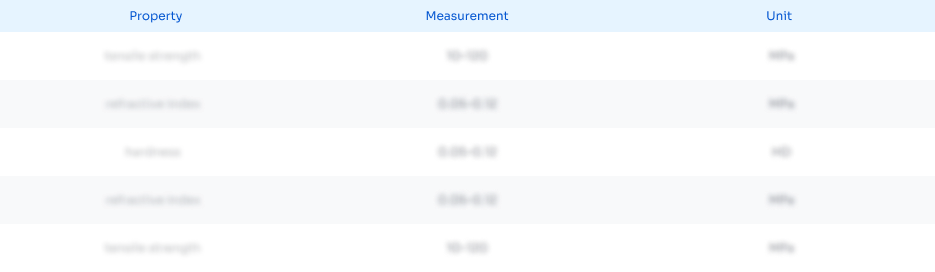
Abstract
Description
Claims
Application Information
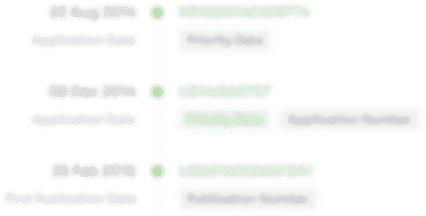
- Generate Ideas
- Intellectual Property
- Life Sciences
- Materials
- Tech Scout
- Unparalleled Data Quality
- Higher Quality Content
- 60% Fewer Hallucinations
Browse by: Latest US Patents, China's latest patents, Technical Efficacy Thesaurus, Application Domain, Technology Topic, Popular Technical Reports.
© 2025 PatSnap. All rights reserved.Legal|Privacy policy|Modern Slavery Act Transparency Statement|Sitemap|About US| Contact US: help@patsnap.com