CT image reconstruction method and system based on convolutional neural network
A convolutional neural network and CT image technology, applied in the medical field, can solve problems such as limiting the application potential of neural networks and not being able to effectively mine useful information of projection data, so as to improve the quality of reconstructed images, improve feature extraction and information expression capabilities, easy-to-achieve effects
- Summary
- Abstract
- Description
- Claims
- Application Information
AI Technical Summary
Problems solved by technology
Method used
Image
Examples
Embodiment Construction
[0021] like figure 1 and figure 2 As shown, the present invention provides a kind of CT image reconstruction method based on convolutional neural network, and this method is applied to CT system, and described method comprises the following steps:
[0022] S1. Obtain projection data;
[0023] S2. Using a front-end convolutional neural network to filter the projection data;
[0024] S3. Perform back-projection processing on the projection data filtered by the front-end convolutional neural network to generate tomographic image information;
[0025] S4. Using the back-end convolutional neural network to process the tomographic image information generated by the back-projection module;
[0026] S5. Calculate the value of the loss function of the entire network by using the tomographic image processed by the back-end convolutional neural network and the real image, and feed back the gradient information of the loss function to the back-end convolutional neural network;
[002...
PUM
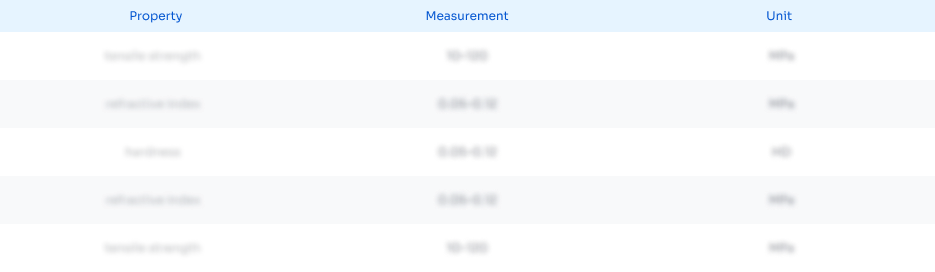
Abstract
Description
Claims
Application Information
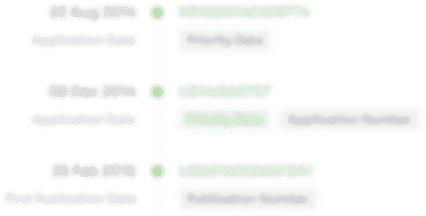
- R&D Engineer
- R&D Manager
- IP Professional
- Industry Leading Data Capabilities
- Powerful AI technology
- Patent DNA Extraction
Browse by: Latest US Patents, China's latest patents, Technical Efficacy Thesaurus, Application Domain, Technology Topic, Popular Technical Reports.
© 2024 PatSnap. All rights reserved.Legal|Privacy policy|Modern Slavery Act Transparency Statement|Sitemap|About US| Contact US: help@patsnap.com