Knowledge representation learning framework based on multi-class cross entropy comparison completion coding
A technology of knowledge representation and cross-entropy, applied in the field of knowledge representation learning framework, can solve problems such as sample imbalance, unlearnable scoring function, and limited representation learning effect, so as to save manpower
- Summary
- Abstract
- Description
- Claims
- Application Information
AI Technical Summary
Problems solved by technology
Method used
Image
Examples
Embodiment Construction
[0028] In order to make the characteristics of the knowledge representation learning framework based on multi-class cross-entropy comparison and completion coding proposed in the present invention more clear, the advantages of the designed automatic completion triplet task and knowledge representation learning method are more obvious. The following is combined with the accompanying drawings and specific implementation methods are described in further detail.
[0029] First, the basic symbol definition of the knowledge map is defined, and the knowledge map is defined as is a collection of entities, is a set of relations, is a set of triplets, is the set of inverse relations, any triple r -1 is the inverse relation of r, so The semantic structure feature extraction function is e(·).
[0030] figure 1It is a schematic diagram of the process of contrastive learning multi-class cross-entropy mutual information estimation proposed by the present invention. It is ill...
PUM
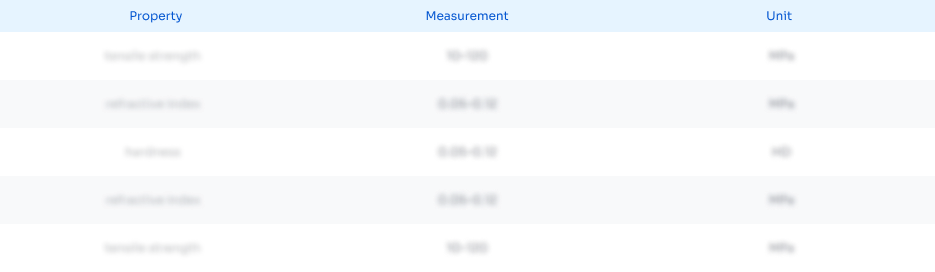
Abstract
Description
Claims
Application Information
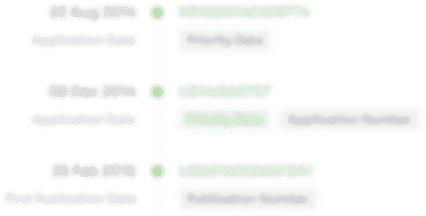
- R&D
- Intellectual Property
- Life Sciences
- Materials
- Tech Scout
- Unparalleled Data Quality
- Higher Quality Content
- 60% Fewer Hallucinations
Browse by: Latest US Patents, China's latest patents, Technical Efficacy Thesaurus, Application Domain, Technology Topic, Popular Technical Reports.
© 2025 PatSnap. All rights reserved.Legal|Privacy policy|Modern Slavery Act Transparency Statement|Sitemap|About US| Contact US: help@patsnap.com