Experience playback sampling reinforcement learning method and system based on confidence upper bound thought
A technology of reinforcement learning and experience, applied in the field of reinforcement learning, can solve problems such as limiting the scope of application, achieve the effect of improving sampling efficiency, improving sampling efficiency and sample utilization, and improving exploration ability
- Summary
- Abstract
- Description
- Claims
- Application Information
AI Technical Summary
Problems solved by technology
Method used
Image
Examples
Embodiment 1
[0077] This embodiment discloses an experience playback sampling reinforcement learning method based on the belief upper bound idea, which includes the following steps: collect the experience obtained by the interaction between the agent and the environment, and store the experience data in the experience playback pool; update the current During the training strategy, randomly select λ·K pieces of experience from the experience playback pool according to the priority probability to generate a candidate training sample set; select the training sample set according to the confidence upper bound value of each candidate training sample; The data updates the parameters of the neural network used for function approximation.
[0078] In specific implementation examples, the purpose of the present invention is achieved through the following technical solutions:
[0079] Such as figure 2 As shown, an experience replay sampling reinforcement learning strategy based on the belief upper...
Embodiment 3
[0133] The purpose of this embodiment is to provide a computing device, including a memory, a processor, and a computer program stored on the memory and operable on the processor. step.
Embodiment 4
[0135] The purpose of this embodiment is to provide a computer-readable storage medium.
[0136] A computer-readable storage medium, on which a computer program is stored, and when the program is executed by a processor, specific steps in the methods of the above-mentioned implementation examples are executed.
PUM
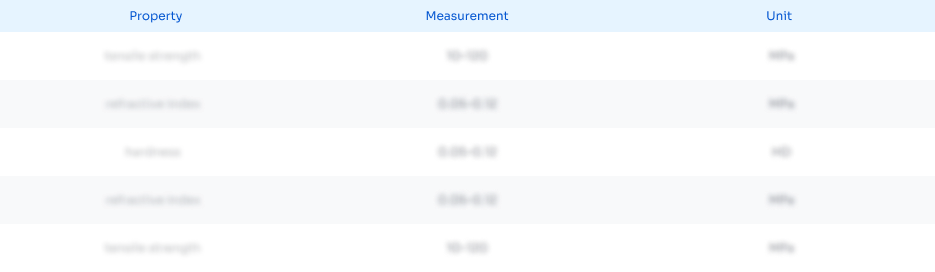
Abstract
Description
Claims
Application Information
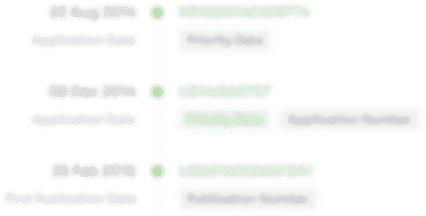
- R&D Engineer
- R&D Manager
- IP Professional
- Industry Leading Data Capabilities
- Powerful AI technology
- Patent DNA Extraction
Browse by: Latest US Patents, China's latest patents, Technical Efficacy Thesaurus, Application Domain, Technology Topic, Popular Technical Reports.
© 2024 PatSnap. All rights reserved.Legal|Privacy policy|Modern Slavery Act Transparency Statement|Sitemap|About US| Contact US: help@patsnap.com