Random survival forest-based postoperative liver cancer recurrence prediction method based on and storage medium
A prediction method and forest algorithm technology, applied in the field of bioinformatics, can solve the problems of difficult to deal with medical data, deviation, low accuracy and so on
- Summary
- Abstract
- Description
- Claims
- Application Information
AI Technical Summary
Problems solved by technology
Method used
Image
Examples
Embodiment 1
[0081] This embodiment provides a method for predicting postoperative recurrence of liver cancer based on random survival forest, which may include the following steps:
[0082] S1: Obtain each case that was enrolled in the group. The preoperative liver function evaluation of each case was normal, there was no previous history of malignant tumor, no adjacent organ invasion and distant metastasis, liver cancer resection was performed, and postoperative pathology confirmed hepatocytes cancer, and recurrence after surgery;
[0083] S2: Obtain the clinical data and recurrence time of each case;
[0084] S3: The preset enrollment dimensions include basic patient factors, preoperative examination factors and postoperative pathological factors;
[0085] Specifically, the basic patient factors include age and gender; the preoperative test factors include platelets, albumin, total bilirubin, etiological examination results, and alpha-fetoprotein; the postoperative pathological factors...
Embodiment 2
[0094] Please refer to figure 2 , this embodiment further defines on the basis of embodiment one:
[0095] The S5 specifically includes:
[0096] S51: Divide each case according to a preset ratio to obtain a training group case and a test group case;
[0097] S52: Divide the data set according to the cases in the training group and the test group to obtain a data set in the training group and a data set in the test group;
[0098] S53: According to the data set of the training group and the recurrence time of each case in the training group, the random survival forest algorithm is used to construct the corresponding early recurrence prediction model of liver cancer and its cumulative risk function;
[0099] S54: Use the cumulative risk function to predict each case in the training group of cases, and obtain a risk score set composed of the risk scores of each case;
[0100] S55: Divide the risk score set according to a preset ratio to obtain ranges of risk scores respectiv...
Embodiment 3
[0109] This embodiment corresponds to Embodiment 2, and the overall scheme is further limited, which can also be referred to figure 2 , methods include:
[0110] S1: Obtain the enrolled cases, and each case must meet the following conditions: normal preoperative liver function assessment, no previous history of malignant tumors, no adjacent organ invasion and distant metastasis, liver cancer resection, and postoperative pathologically confirmed hepatocytes Cancer, and recurrence after surgery;
[0111] S2: Obtain the recurrence time, relevant clinical data and follow-up data of each of the above cases, and exclude patients with incomplete data;
[0112] S3: Determine the inclusion dimensions, including at least:
[0113] 1. Basic factors of the case: gender, age;
[0114] 2. Preoperative inspection factors: platelets, albumin, total bilirubin, etiological examination (hepatitis B, hepatitis C, others), alpha-fetoprotein;
[0115] 3. Postoperative pathological factors: max...
PUM
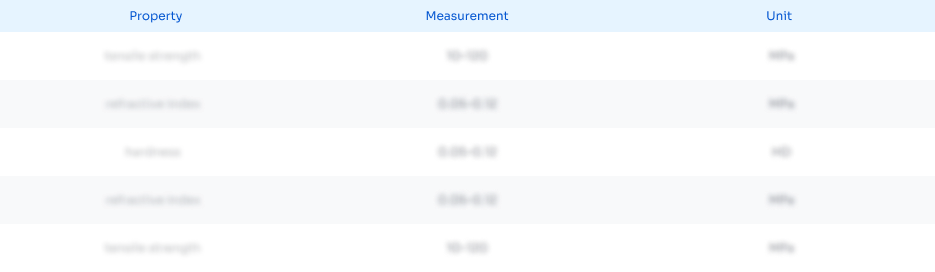
Abstract
Description
Claims
Application Information
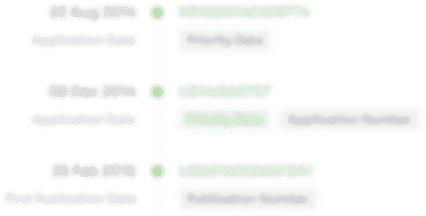
- R&D Engineer
- R&D Manager
- IP Professional
- Industry Leading Data Capabilities
- Powerful AI technology
- Patent DNA Extraction
Browse by: Latest US Patents, China's latest patents, Technical Efficacy Thesaurus, Application Domain, Technology Topic, Popular Technical Reports.
© 2024 PatSnap. All rights reserved.Legal|Privacy policy|Modern Slavery Act Transparency Statement|Sitemap|About US| Contact US: help@patsnap.com