Hybrid pipeline parallel method for accelerating distributed deep neural network training
A deep neural network and neural network technology, applied in the field of hybrid model division and task placement, can solve problems such as training acceleration, and achieve the effect of increasing possibilities and improving training speed.
- Summary
- Abstract
- Description
- Claims
- Application Information
AI Technical Summary
Problems solved by technology
Method used
Image
Examples
Embodiment 1
[0030] Embodiment 1: see figure 1 , a hybrid pipeline parallel method for accelerating distributed deep neural network training, the method includes the following steps:
[0031] Step 1: Establish the hierarchical cumulative distribution function (CDF) model of the deep neural network, analyze the corresponding input conditions required by the deep learning application execution model division and task placement algorithm, and use the pytorch framework to obtain the parameter amount of each layer; then according to the given The batch-size size of each layer is used to calculate the intermediate result traffic of each layer; finally, according to the type of each layer of the network, such as convolutional layer, fully connected layer, etc., calculate the floating point calculation amount of each layer, and do it for step 2 Preparation;
[0032]Step 2: According to the result of step 1, use the dynamic programming algorithm to solve the parallel time between any two layers of...
specific Embodiment
[0046] Specific embodiments: the present invention is mainly carried out in a GPU cluster environment.
[0047] figure 1 It shows a schematic diagram of a GPU cluster, which mainly includes several GPU server nodes. Each server node has several GPUs. The nodes are connected through PCIe, and the nodes are connected through Ethernet.
[0048] figure 2 Represents a schematic diagram of the hybrid parallel method. The same rectangle is divided into two parts, representing the division method, and the rectangles of different parts are stacked together to represent the repeated calculation part, that is, the part that needs communication. The three graphs correspond to three tensor division methods, which require communication weight parameters, output layer data, and input layer data respectively.
[0049] image 3 Represents the overall flow chart, the first choice is to initialize the system, load the input data and create a specified model, then describe the model by layer,...
PUM
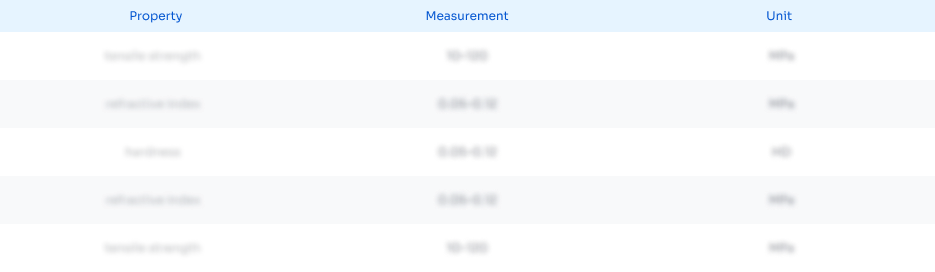
Abstract
Description
Claims
Application Information
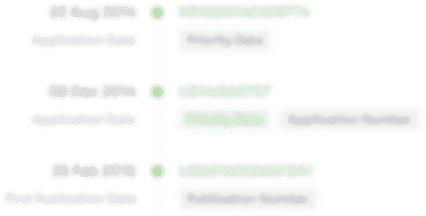
- R&D
- Intellectual Property
- Life Sciences
- Materials
- Tech Scout
- Unparalleled Data Quality
- Higher Quality Content
- 60% Fewer Hallucinations
Browse by: Latest US Patents, China's latest patents, Technical Efficacy Thesaurus, Application Domain, Technology Topic, Popular Technical Reports.
© 2025 PatSnap. All rights reserved.Legal|Privacy policy|Modern Slavery Act Transparency Statement|Sitemap|About US| Contact US: help@patsnap.com