Alzheimer's disease classification method based on multi-modal hypergraph convolutional neural network
A convolutional neural network and Alzheimer's disease technology, applied in the field of intelligent medical computer-aided diagnosis, can solve problems such as complex structure and complex pair relationship, and achieve the effect of shortening time, high classification effect, and small data dimension
- Summary
- Abstract
- Description
- Claims
- Application Information
AI Technical Summary
Problems solved by technology
Method used
Image
Examples
Embodiment 1
[0077] In accordance with the implementation of the training phase, the examples are done for the data in the standard dataset ADNI database. The data of subjects with three modalities of MRI, PET and CSF at the same time were selected for the experiment, and only the data collected at the fiducial points of these subjects were selected. In the ADNI database, there are 202 subjects with the above three modalities at the same time, including 51 with Alzheimer's disease (AD), 52 in the normal control group (Normal Control, NC), mild Cognitive Impairment (MCI) has 99. MCI was further divided into 56 patients who did not convert to Alzheimer's disease after 18 months (ie MCI non-converters, MCI-nc), and 56 who converted to Alzheimer's disease after 18 months (ie MCI converters). , MCI-c), was 43. The demographic information of these subjects is shown in Table 1:
[0078] Table 1: ADNI Demographic Information of 202 Subjects with Three Modalities: MRI, PET, and CSF
[0079] ...
Embodiment 2
[0087] In order to verify that the classification effect of multi-modal features is better than that of single-modal features, the present invention also uses different single modalities for classification, compares with the comprehensive multi-modal classification, and finally calculates the classification effects of various modalities and draws them as Table 4, Table 5, Table 6. The feature extraction method and process when using unimodal classification is the same as that of multimodality, and the final classifier uses unimodal TSK fuzzy classifier. It can be seen from the table that no matter what kind of classification task, the multimodal method achieves better results than the single modality. In the classification task of MCI vs. NC, the classification result of combining multiple modalities is 10% higher than the highest result in single-modality classification, reaching 86.83%, and the classification effect of multiple modalities in several other classification task...
PUM
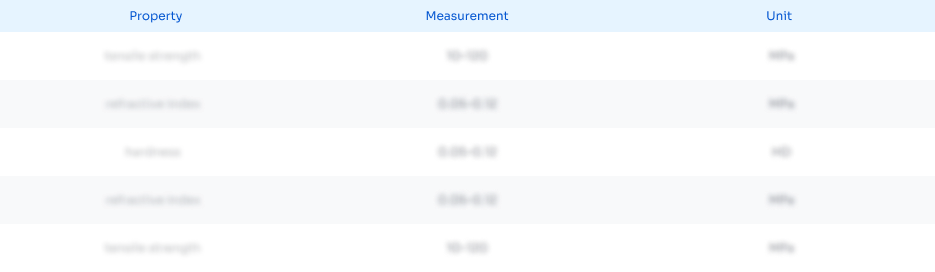
Abstract
Description
Claims
Application Information
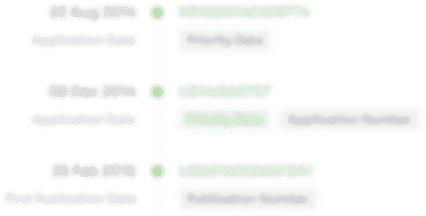
- R&D
- Intellectual Property
- Life Sciences
- Materials
- Tech Scout
- Unparalleled Data Quality
- Higher Quality Content
- 60% Fewer Hallucinations
Browse by: Latest US Patents, China's latest patents, Technical Efficacy Thesaurus, Application Domain, Technology Topic, Popular Technical Reports.
© 2025 PatSnap. All rights reserved.Legal|Privacy policy|Modern Slavery Act Transparency Statement|Sitemap|About US| Contact US: help@patsnap.com