Deep learning algorithm for detecting anomaly and segmenting abnormal region in image
An anomaly area and anomaly detection technology, applied in the field of unsupervised image anomaly detection and segmentation, can solve problems such as poor reconstruction effect of complex patterns, large amount of model parameters, and long training time
- Summary
- Abstract
- Description
- Claims
- Application Information
AI Technical Summary
Problems solved by technology
Method used
Image
Examples
Embodiment Construction
[0026] The technical solutions in the embodiments of the present invention will be clearly and completely described below in conjunction with the embodiments of the present invention. Obviously, the described embodiments are only part of the embodiments of the present invention, not all of them. Based on the present invention All other embodiments obtained by persons of ordinary skill in the art without creative efforts fall within the protection scope of the present invention.
[0027] The invention provides a technical solution:
[0028] A deep learning algorithm for detecting abnormalities and segmenting abnormal regions in an image, comprising the following steps:
[0029] S1, first extract the features of the positive sample image, use the classification network ViT (visual transformer) pre-training model based on the large-scale public data set (ImageNet) to extract features from the positive sample training set, and the obtained data body dimension is expressed as (N ,...
PUM
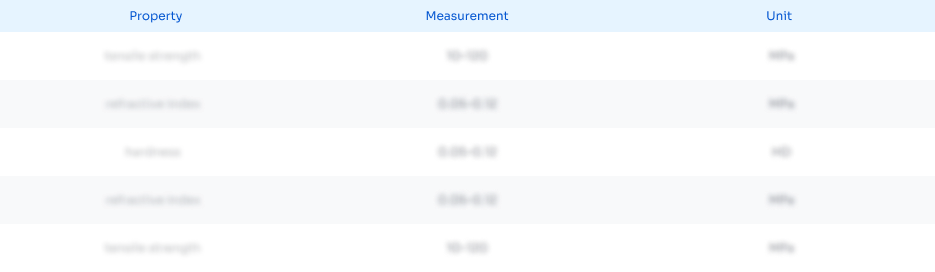
Abstract
Description
Claims
Application Information
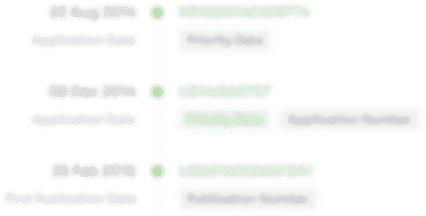
- R&D Engineer
- R&D Manager
- IP Professional
- Industry Leading Data Capabilities
- Powerful AI technology
- Patent DNA Extraction
Browse by: Latest US Patents, China's latest patents, Technical Efficacy Thesaurus, Application Domain, Technology Topic, Popular Technical Reports.
© 2024 PatSnap. All rights reserved.Legal|Privacy policy|Modern Slavery Act Transparency Statement|Sitemap|About US| Contact US: help@patsnap.com