Large-scale edge machine learning training method based on probabilistic sampling
A technology of learning training and machine learning, applied in machine learning, instruments, computing models, etc., can solve problems such as unguaranteed algorithm convergence and reduced model accuracy, and achieve the goals of increasing scalability, ensuring accuracy, and improving accuracy Effect
- Summary
- Abstract
- Description
- Claims
- Application Information
AI Technical Summary
Problems solved by technology
Method used
Image
Examples
Embodiment Construction
[0036] In order to make the object, technical scheme and advantages of the present invention clearer, the following in conjunction with the attached Figure 1-3 The specific embodiment of the learning and training method of the present invention is further described in detail.
[0037] Through probabilistic node selection, the progress of each node in distributed computing is only determined by a certain subset of the overall nodes, thereby reducing communication overhead and improving the overall progress of the system while ensuring that the calculation accuracy is only limited. For this trade-off, in order to quantitatively investigate the impact of this method on system performance, it is necessary to analyze the convergence of the probabilistic node selection method based on mathematical modeling. The method of the present invention is based on the convergence analysis of the existing synchronization method , the inconsistency (inconsistency) R[X] in the distributed syste...
PUM
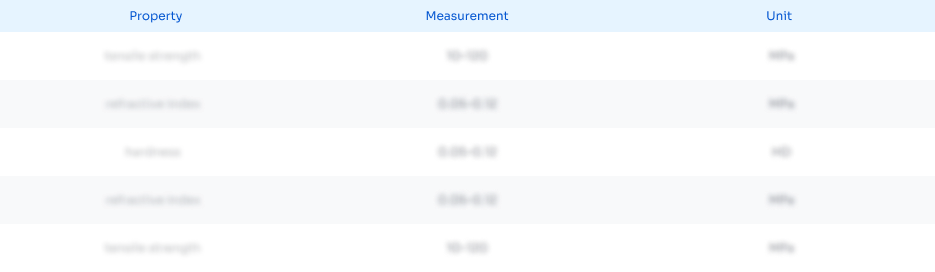
Abstract
Description
Claims
Application Information
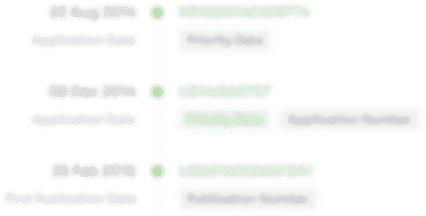
- R&D Engineer
- R&D Manager
- IP Professional
- Industry Leading Data Capabilities
- Powerful AI technology
- Patent DNA Extraction
Browse by: Latest US Patents, China's latest patents, Technical Efficacy Thesaurus, Application Domain, Technology Topic.
© 2024 PatSnap. All rights reserved.Legal|Privacy policy|Modern Slavery Act Transparency Statement|Sitemap