Cross-modal retrieval method based on deep self-supervised sorting hash
A cross-modal and deep technology, applied in the field of pattern recognition, can solve the problems of large coding error, poor retrieval performance, unsatisfactory retrieval performance, etc., and achieve the effect of good robustness
- Summary
- Abstract
- Description
- Claims
- Application Information
AI Technical Summary
Problems solved by technology
Method used
Image
Examples
Embodiment Construction
[0037] The technical solution of the present invention will be further described in detail in conjunction with the accompanying drawings and specific implementation: the present invention provides a cross-modal retrieval algorithm based on deep self-supervised sorting hash, and the specific process is as follows figure 1 shown.
[0038] Step (1): Obtain a training dataset, where each sample includes text, images and labels. Here we use three widely used benchmark multimodal datasets, namely Wiki, MIRFlickr and NUS-WIDE.
[0039] Step (2): Use the label information to train the label network. The specific method is:
[0040] The purpose of label network is to learn the semantic features of instances to guide the feature learning of image and text networks. Semantic feature learning: Using a 4-layer fully connected network, the input layer of the neural network is the label of the instance, the second layer has 4096 nodes, uses the Relu activation function and performs local n...
PUM
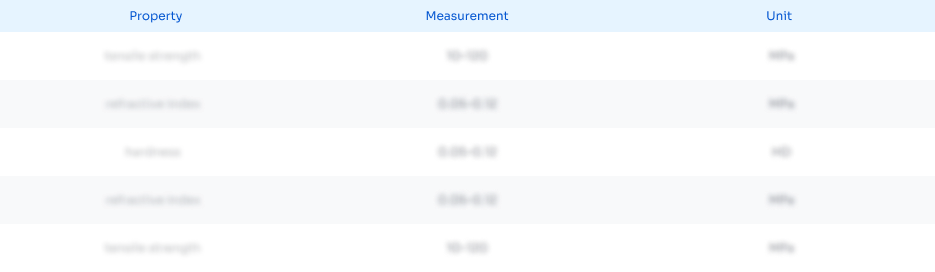
Abstract
Description
Claims
Application Information
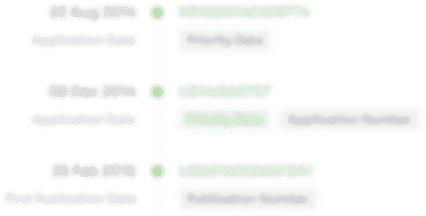
- R&D Engineer
- R&D Manager
- IP Professional
- Industry Leading Data Capabilities
- Powerful AI technology
- Patent DNA Extraction
Browse by: Latest US Patents, China's latest patents, Technical Efficacy Thesaurus, Application Domain, Technology Topic, Popular Technical Reports.
© 2024 PatSnap. All rights reserved.Legal|Privacy policy|Modern Slavery Act Transparency Statement|Sitemap|About US| Contact US: help@patsnap.com