Rolling bearing fault diagnosis method based on SSA-WDCNN
A technology for rolling bearings and fault diagnosis, which is applied in neural learning methods, testing of mechanical components, recognition of patterns in signals, etc. It can solve the problem of limited use range, small signal-to-noise ratio signals, large noise signals, and the characteristics are not ideal, and fault signals are easy to fail. Diagnosis deviation and other problems occur, to achieve the effect of widening the scope, ensuring the practical application ability, and good signal noise reduction effect
- Summary
- Abstract
- Description
- Claims
- Application Information
AI Technical Summary
Problems solved by technology
Method used
Image
Examples
Embodiment
[0051] A rolling bearing fault diagnosis method based on SSA-WDCNN, comprising the following steps:
[0052] S1: Singular spectrum analysis is performed on the vibration signal of rolling bearing with noise to obtain the reconstructed vibration signal.
[0053] Step S1 specifically includes:
[0054] S11: Transform the noise-containing rolling bearing vibration signal into a trajectory matrix.
[0055] The noise-containing rolling bearing vibration signal X in step S11 N for:
[0056] x N ={f 1 ,f 2 ,..., f N}
[0057] Among them, f N is a time series signal component of length N,
[0058] The transformed trajectory matrix X is:
[0059]
[0060] Among them, f n+m-1 is the element signal of the m×n window trajectory matrix.
[0061] S12: Perform singular value decomposition on the trajectory matrix.
[0062] The specific steps of step S12 include:
[0063] Define the covariance matrix C=XX T , find the eigenvalues of the matrix C and arrange them in descendin...
PUM
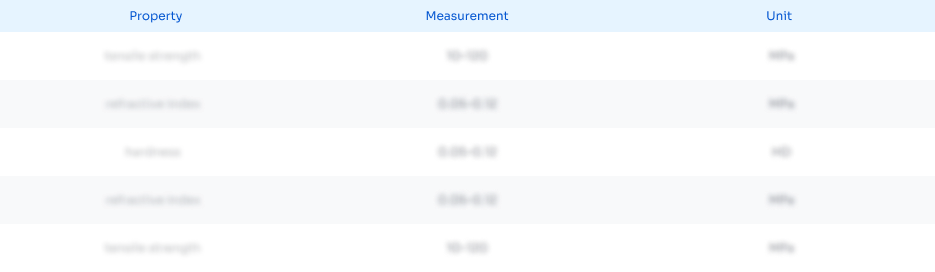
Abstract
Description
Claims
Application Information
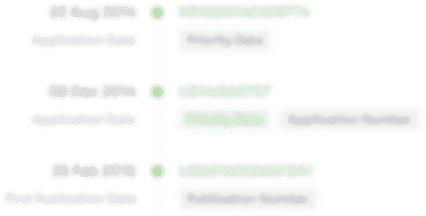
- R&D Engineer
- R&D Manager
- IP Professional
- Industry Leading Data Capabilities
- Powerful AI technology
- Patent DNA Extraction
Browse by: Latest US Patents, China's latest patents, Technical Efficacy Thesaurus, Application Domain, Technology Topic, Popular Technical Reports.
© 2024 PatSnap. All rights reserved.Legal|Privacy policy|Modern Slavery Act Transparency Statement|Sitemap|About US| Contact US: help@patsnap.com