Hypergraph-based drug-target-disease interaction prediction method
A prediction method and disease technology, applied in the field of prediction of drug-target-disease interaction relationship, can solve the problems of insufficient description of data relationship, emphasizing, ignoring dependencies, etc.
- Summary
- Abstract
- Description
- Claims
- Application Information
AI Technical Summary
Problems solved by technology
Method used
Image
Examples
Embodiment Construction
[0029] The detailed method flow process of the present invention is further described below:
[0030] The invention provides a drug-target-disease interaction prediction method based on a hypergraph neural network, which uses a hypergraph neural network and a graph convolutional network to extract entity relationship information, and automatically learns node feature representations for relationship prediction through deep model training. The steps include: building semantic hypergraph and feature similarity graph, updating node representation, representation fusion, potential relationship prediction. The present invention overcomes the limitations of most methods that only model binary relationships such as drug-target or drug-disease, effectively models high-order relationships and dependencies between medical data, and improves the prediction of drug-target-disease interaction relationships Accuracy to facilitate drug discovery research.
[0031] Such as figure 1 The flow...
PUM
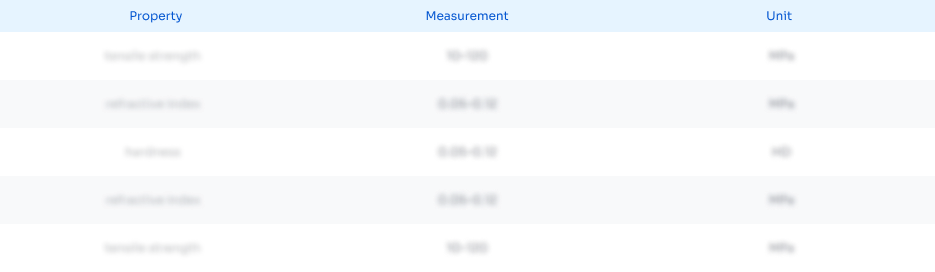
Abstract
Description
Claims
Application Information
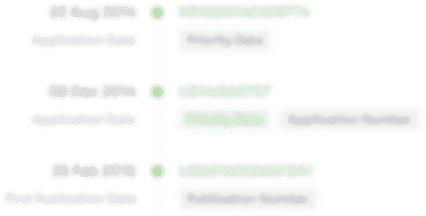
- R&D Engineer
- R&D Manager
- IP Professional
- Industry Leading Data Capabilities
- Powerful AI technology
- Patent DNA Extraction
Browse by: Latest US Patents, China's latest patents, Technical Efficacy Thesaurus, Application Domain, Technology Topic.
© 2024 PatSnap. All rights reserved.Legal|Privacy policy|Modern Slavery Act Transparency Statement|Sitemap