Convolutional neural network training method based on network image
A convolutional neural network, network image technology, applied in neural learning methods, biological neural network models, neural architectures, etc., can solve the problems of limited number of images, accuracy can not reach the standard, and recognition model recognition categories are insufficient.
- Summary
- Abstract
- Description
- Claims
- Application Information
AI Technical Summary
Problems solved by technology
Method used
Image
Examples
Embodiment 1
[0054] Example 1: Web image crawling
[0055] It is difficult to label the brand of the vehicle captured in the surveillance image, and at the same time, there are vehicle images with good brand label information on the Internet. It is hoped that the brand-labeled image of the network will be used as the initial data for the training of the vehicle brand recognition model. Therefore, it is first necessary to crawl the high-quality labeled vehicle images on the Internet. Crawl the image data of vehicles marked with vehicle brands by crawling pictures on autolist.com, autohome.com and other websites such as automobile information and second-hand car sales networks.
Embodiment 2
[0056] Example 2: Iterative data screening and model training
[0057] Due to the great difference between the network image and the surveillance image (the feature distribution is inconsistent), it is not good to directly use the model trained by the network image to recognize the surveillance image, so a gradual data screening and model training is adopted. method. For the initial stage, network images are used as the training set to train a vehicle brand recognition model, which is then applied to surveillance images. For each input image, the recognition model outputs a class probability distribution, where a value in the distribution represents the probability that the image contains a number of vehicles belonging to a certain class. The image is filtered according to a preset threshold. Only keep images with the largest value in the distribution greater than a preset threshold. For these retained images, the category represented by the maximum value is also added to t...
Embodiment 3
[0058] Embodiment 3: basic feature extraction
[0059] For an image that contains the back shot of the vehicle, the size of the image is adjusted to 256*256 by linear interpolation, and then input into the convolutional neural network, and the output of the model is aggregated through a layer of convolutional network layers. The output of this layer is the extracted image representation. The representation is a one-dimensional vector with 2048 elements.
PUM
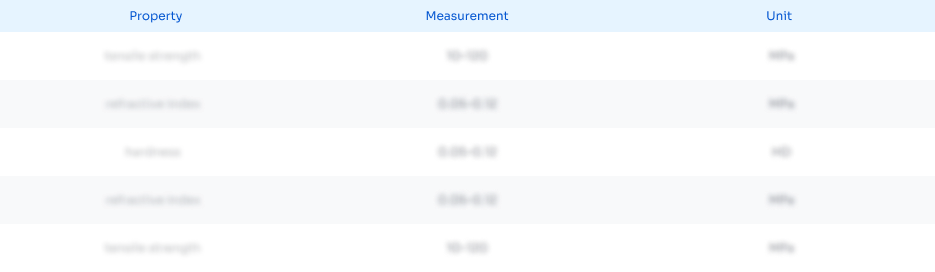
Abstract
Description
Claims
Application Information
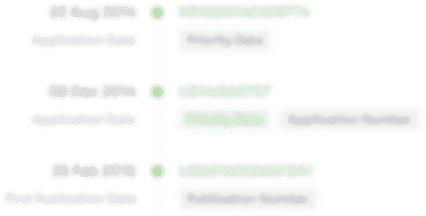
- R&D Engineer
- R&D Manager
- IP Professional
- Industry Leading Data Capabilities
- Powerful AI technology
- Patent DNA Extraction
Browse by: Latest US Patents, China's latest patents, Technical Efficacy Thesaurus, Application Domain, Technology Topic, Popular Technical Reports.
© 2024 PatSnap. All rights reserved.Legal|Privacy policy|Modern Slavery Act Transparency Statement|Sitemap|About US| Contact US: help@patsnap.com