Data-augmented deep semi-supervised extreme learning image classification method and system
A classification method and semi-supervised technology, applied in the field of target recognition and image classification, can solve the problems of low utilization efficiency of sample data information, difficult learning and training, difficult classifiers, etc.
- Summary
- Abstract
- Description
- Claims
- Application Information
AI Technical Summary
Problems solved by technology
Method used
Image
Examples
Embodiment Construction
[0045] The present invention will be further described below in conjunction with the accompanying drawings and specific preferred embodiments, but the protection scope of the present invention is not limited thereby.
[0046] Such as figure 1 and figure 2 As shown, the deep semi-supervised over-limit learning image classification method of data augmentation in this embodiment includes the following steps of learning and training the image classification recognition network model:
[0047] S1. Use the deep convolutional network model for feature extraction for the training image; train the deep convolutional network model for some artificially labeled data of the training image to achieve fine-tuning optimization, and the deep convolutional network model after fine-tuning optimization is unlabeled The training image predictions generate corresponding pseudo-labels;
[0048] S2. Fusing high-level semantic features extracted from training images with low-level shallow structu...
PUM
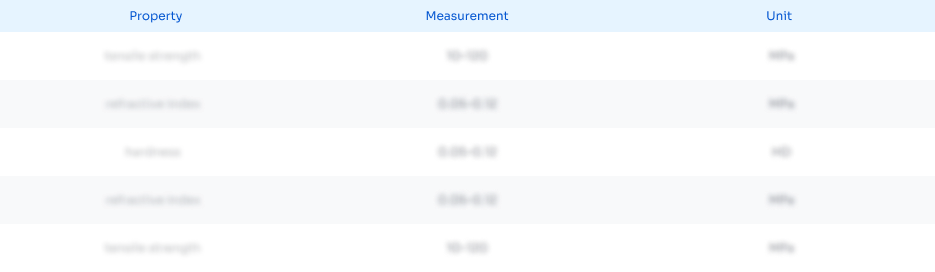
Abstract
Description
Claims
Application Information
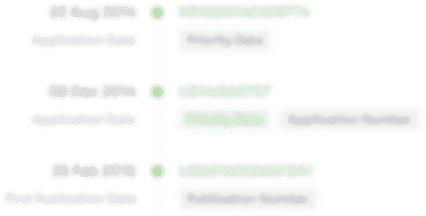
- R&D Engineer
- R&D Manager
- IP Professional
- Industry Leading Data Capabilities
- Powerful AI technology
- Patent DNA Extraction
Browse by: Latest US Patents, China's latest patents, Technical Efficacy Thesaurus, Application Domain, Technology Topic, Popular Technical Reports.
© 2024 PatSnap. All rights reserved.Legal|Privacy policy|Modern Slavery Act Transparency Statement|Sitemap|About US| Contact US: help@patsnap.com