Artificial intelligence data labeling method and device
An artificial intelligence and data technology, applied in the field of data processing, can solve the problems that the scale of annotation is difficult to keep consistent, the subjective influence of annotators and reviewers is large, and the accuracy is not high. The effect of labeling errors
- Summary
- Abstract
- Description
- Claims
- Application Information
AI Technical Summary
Problems solved by technology
Method used
Image
Examples
Embodiment 1
[0066] see figure 2 , figure 2 It is a schematic diagram of the artificial intelligence data labeling process in the embodiment of this application. The specific steps are:
[0067] Step 201, acquiring a data set to be labeled.
[0068] Step 202, based on the established AI model, obtain the AI label with the highest probability score for each piece of data to be labeled, and the corresponding probability score.
[0069] In specific implementation, one or more established AI models can also be used to obtain the AI label with the highest probability score for each piece of data to be labeled and the corresponding probability score.
[0070] Taking M AI models as an example, obtain the AI label with the highest probability score for each piece of data to be labeled based on the established AI model, as well as the probability score, including:
[0071] Obtain the probability score corresponding to each AI label corresponding to the model based on the established M A...
Embodiment 2
[0089] see image 3 , image 3 It is a schematic flowchart of using the data marked by the AI model as the data sample for training the AI model in the embodiment of the present application. The specific steps are:
[0090] Step 301, acquiring a data set to be labeled.
[0091] Step 302, based on the established AI model, obtain the AI label with the highest probability score for each piece of data to be labeled, and the corresponding probability score.
[0092] Step 303, for any data to be labeled, determine whether the probability score is greater than a first preset threshold.
[0093] Step 304, when it is determined that the probability score is greater than the first preset threshold and it is determined to sample the data to be labeled, label the data to be labeled with a manual label.
[0094] Step 305, determine whether the artificial label is consistent with the acquired AI label, if yes, perform step 309; otherwise, perform step 308.
[0095] Step 306, whe...
Embodiment 3
[0103] see Figure 4 , Figure 4 It is a schematic flowchart of determining whether to update the first threshold according to the accuracy rate in the embodiment of the present application. The specific steps are:
[0104] Step 401, acquiring a data set to be labeled.
[0105] Step 402, based on the established AI model, obtain the AI label with the highest probability score for each piece of data to be labeled, and the corresponding probability score.
[0106] Step 403, for any data to be labeled, determine whether the probability score is greater than a first preset threshold.
[0107] Step 404, when it is determined that the probability score is greater than the first preset threshold, and it is determined to spot check the data to be labeled, and label the data to be labeled with a manual label; and record whether the manual label for the data is consistent with the acquired AI label, Execute step 406.
[0108] Step 405, when it is determined that the probability s...
PUM
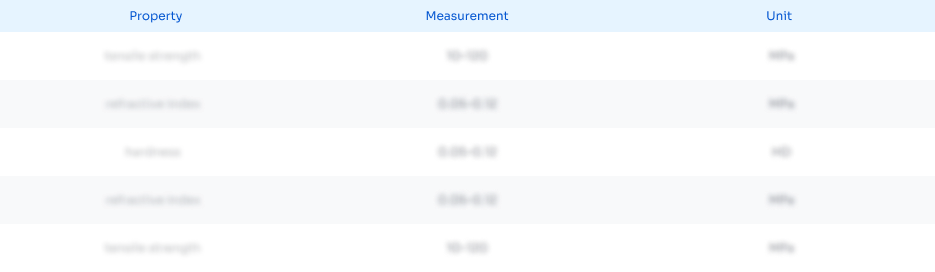
Abstract
Description
Claims
Application Information
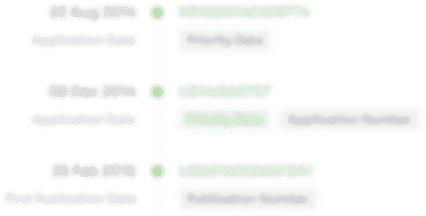
- R&D Engineer
- R&D Manager
- IP Professional
- Industry Leading Data Capabilities
- Powerful AI technology
- Patent DNA Extraction
Browse by: Latest US Patents, China's latest patents, Technical Efficacy Thesaurus, Application Domain, Technology Topic, Popular Technical Reports.
© 2024 PatSnap. All rights reserved.Legal|Privacy policy|Modern Slavery Act Transparency Statement|Sitemap|About US| Contact US: help@patsnap.com