Spore and spore identification method and system based on residual deep network, and medium
A deep network and spore spore technology, applied in the field of deep learning, can solve the problems of high labor intensity, high degree of subjective judgment of results, missed and false positives of test specimens, etc., to reduce the rate of misdiagnosis and missed diagnosis, excellent generalization ability, The effect of reducing business capability requirements
- Summary
- Abstract
- Description
- Claims
- Application Information
AI Technical Summary
Problems solved by technology
Method used
Image
Examples
Embodiment 1
[0041] According to the spore spore recognition method based on the residual deep network provided by the present invention, it includes: Step 1: Segment the original picture collected from the automatic biological microscope using a morphological method, and screen the target pictures that need to be identified to form a sample set and mark them ; Step 2: Perform data amplification on the marked sample set to form the final training data set, and divide the training data set into training set, verification set and test set; Step 3: Build a deep residual network on the caffe framework and set the super Parameters, use the data set for training to obtain the deep residual network training model; Step 4: After performing morphological segmentation on the original pictures collected in real time, use the deep residual network training model with the highest comprehensive recognition rate after the test to perform recognition scoring, and the The result greater than the confidence ...
Embodiment 2
[0047] Embodiment 2 is a preferred example of Embodiment 1.
[0048] Such as figure 1 Shown is a schematic flow chart of a method for identifying spores and spores based on a residual depth network provided by an embodiment of the present invention, including:
[0049] Step 1: Manually screen and mark the segmented single target, including target category and interference category;
[0050] Step 2: After the marked samples are divided into categories, use data enhancement methods such as flipping, translation, cropping, and contrast adjustment to perform data amplification;
[0051] Step 3: Divide the amplified data set into training set, verification set and test set according to the ratio of 7:2:1;
[0052] Step 4: Use convert_imageset in the caffe framework to create the LMDB data file to convert the data format; use the compute_image_mean.cpp file provided in the caffe framework to generate the mean file for training;
[0053] Step 5: Construct the residual neural netwo...
PUM
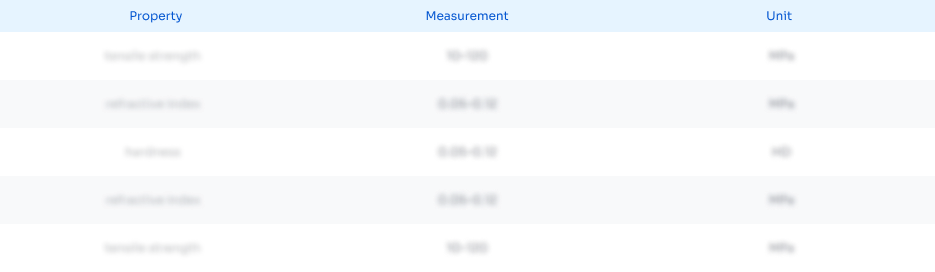
Abstract
Description
Claims
Application Information
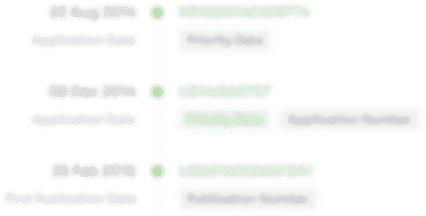
- R&D
- Intellectual Property
- Life Sciences
- Materials
- Tech Scout
- Unparalleled Data Quality
- Higher Quality Content
- 60% Fewer Hallucinations
Browse by: Latest US Patents, China's latest patents, Technical Efficacy Thesaurus, Application Domain, Technology Topic, Popular Technical Reports.
© 2025 PatSnap. All rights reserved.Legal|Privacy policy|Modern Slavery Act Transparency Statement|Sitemap|About US| Contact US: help@patsnap.com