Mobile phone shell rear cover defect detection method and system based on deep learning
A mobile phone case, deep learning technology, applied in the field of visual detection, to achieve the effect of high detection accuracy, fast speed and strong robustness
- Summary
- Abstract
- Description
- Claims
- Application Information
AI Technical Summary
Problems solved by technology
Method used
Image
Examples
specific Embodiment approach 1
[0063] refer to Figure 1-2 as shown,
[0064] A method for detecting defects of a mobile phone case back cover based on deep learning, comprising the following steps:
[0065] Step 1: the image acquisition module acquires images;
[0066] Step 2: Select different types of defective image blocks and non-defective image blocks as training sample sets;
[0067] Step 3: Build a deep learning neural network for learning training and prediction;
[0068] Step 4: Use the training sample offline set to train the deep learning algorithm;
[0069] Step 5: Use the trained deep learning algorithm to detect and identify defects in the surface shell image of the mobile phone online.
[0070] Said step 3 includes:
[0071] Step 3.1: Use the selective search algorithm Selective Search for region selection to find out the Region Proposal of the candidate region where the target may exist in the picture;
[0072] Step 3.2: Adjust the size of the image to suit the input of the subsequent ...
specific Embodiment approach 2
[0105] refer to Figure 1-3 as shown,
[0106] A method for detecting defects of the back cover of a mobile phone case based on deep learning, and a defect detection system for the back cover of a mobile phone case based on deep learning, including: a mobile phone carrying platform, an image acquisition module, an image processing module, and a control feedback module;
[0107] The mobile phone bearing platform is an automatic translation device for carrying the mobile phone and realizing two-degree-of-freedom plane movement, and realizes plane positioning, bearing and two-degree-of-freedom movement of the mobile phone;
[0108] The mobile phone carrying platform includes a custom positioning fixture 1, a sliding assembly I2 and a sliding assembly II3, the custom positioning fixture 1 is used to place the mobile phone, and the sliding assembly I2 and the sliding assembly II3 drive the custom positioning fixture 1 in a manner that the motor drives the slider to move. The X-axi...
PUM
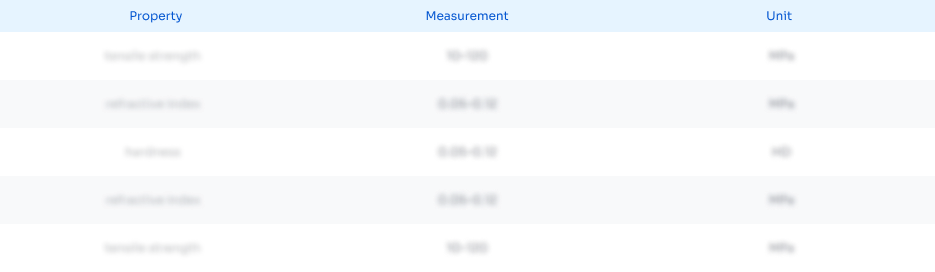
Abstract
Description
Claims
Application Information
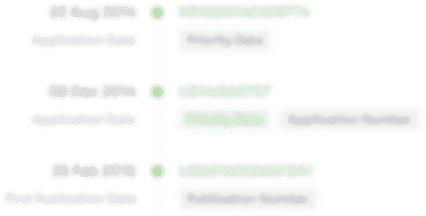
- R&D Engineer
- R&D Manager
- IP Professional
- Industry Leading Data Capabilities
- Powerful AI technology
- Patent DNA Extraction
Browse by: Latest US Patents, China's latest patents, Technical Efficacy Thesaurus, Application Domain, Technology Topic, Popular Technical Reports.
© 2024 PatSnap. All rights reserved.Legal|Privacy policy|Modern Slavery Act Transparency Statement|Sitemap|About US| Contact US: help@patsnap.com