Wood defect detection method based on deep learning and system thereof
A technology of deep learning and defect detection, applied in the field of visual inspection, to achieve the effects of high detection accuracy, fast speed and strong robustness
- Summary
- Abstract
- Description
- Claims
- Application Information
AI Technical Summary
Problems solved by technology
Method used
Image
Examples
Embodiment 1
[0042] Embodiment 1: A wood defect detection method based on deep learning.
[0043] refer to figure 1 As shown, a wood defect detection method based on deep learning, specifically includes the following steps:
[0044] Step 1: The image acquisition module collects images, that is, uses a special light source for machine vision and an industrial camera to collect wood surface images;
[0045] Step 2: Divide the image into image blocks of the same size. The specific segmentation method is: take s / 2 as the step size, divide the collected image into square image blocks with the size of s*s, where the size s generally cannot Smaller than the size of the wood defect, the size of the image block can be 128*128, where 128 represents the pixel size of the side length of the divided image block;
[0046] Step 3: Select different types of defective image blocks and non-defective image blocks as training sample sets;
[0047]Among them, different types of defective image blocks and no...
Embodiment 2
[0060] Example 2: A wood defect detection system based on deep learning
[0061] A wood defect detection system based on deep learning, specifically including:
[0062] An image acquisition module, the image acquisition module is an industrial camera that adopts a linear array CCD, and can utilize a special light source for machine vision and an industrial camera to collect surface images;
[0063] Image processing module, described image processing module is the upper computer software that adopts industrial computer to carry image processing module, and this software includes image processing interactive interface, deep learning algorithm, database and communication module, can utilize deep learning algorithm to carry out wood processing to the image that collects Defect detection, and send the defect information to the control execution unit;
[0064] Control execution module: According to the defect information sent by the image processing module, the programmable logic c...
PUM
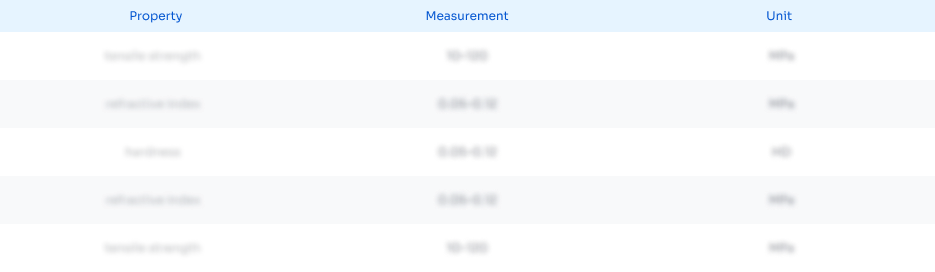
Abstract
Description
Claims
Application Information
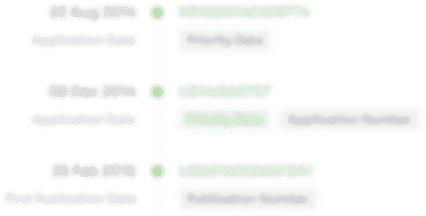
- R&D Engineer
- R&D Manager
- IP Professional
- Industry Leading Data Capabilities
- Powerful AI technology
- Patent DNA Extraction
Browse by: Latest US Patents, China's latest patents, Technical Efficacy Thesaurus, Application Domain, Technology Topic, Popular Technical Reports.
© 2024 PatSnap. All rights reserved.Legal|Privacy policy|Modern Slavery Act Transparency Statement|Sitemap|About US| Contact US: help@patsnap.com