Backdoor confrontation sample generation method of PE malicious software detection model
A technology for detecting models and malware, applied in neural learning methods, biological neural network models, computer components, etc. Robustness, the effect of reducing computational overhead
- Summary
- Abstract
- Description
- Claims
- Application Information
AI Technical Summary
Problems solved by technology
Method used
Image
Examples
Embodiment Construction
[0022] In order to better illustrate the objects and advantages of the present invention, the embodiments of the methods of the invention will be further described in detail below.
[0023] Experimental data from malicious software static feature dataset EMBER2017 and Virusshare.com public PE malware. The EMBER2017 data set contains feature data extracted from 1.1M binary. The training set contains 900K samples, including 300K-based samples, 300K malicious samples, and 300K no label samples; test sets contain 200K samples, including 100K A wellness sample and 100K malicious samples. Public PE malware from Virusshare.com is used to simulate the actual attack effect.
[0024] Table 1PE malware black box to the anti-sample generation experimental data
[0025]
[0026] This experiment is carried out on a computer, and the specific configuration of the computer is: Inter i7-7500U, CPU3.1GHz, memory 8G, operating system is Windows 10.
[0027] The specific process is:
[0028] Step 1...
PUM
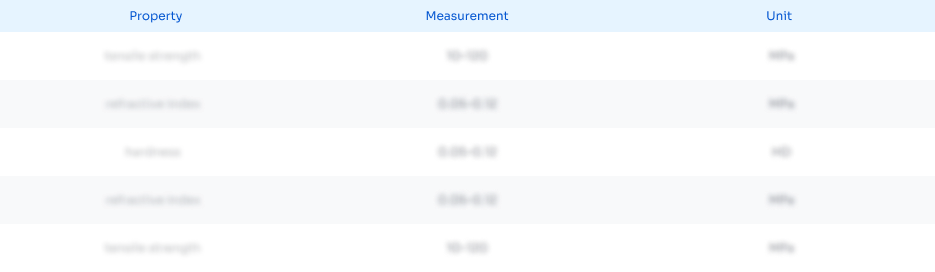
Abstract
Description
Claims
Application Information
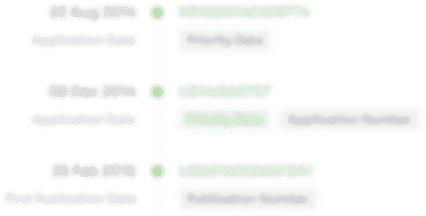
- R&D
- Intellectual Property
- Life Sciences
- Materials
- Tech Scout
- Unparalleled Data Quality
- Higher Quality Content
- 60% Fewer Hallucinations
Browse by: Latest US Patents, China's latest patents, Technical Efficacy Thesaurus, Application Domain, Technology Topic, Popular Technical Reports.
© 2025 PatSnap. All rights reserved.Legal|Privacy policy|Modern Slavery Act Transparency Statement|Sitemap|About US| Contact US: help@patsnap.com