Equipment fault early warning method and system based on multi-modal sensitive feature selection fusion
A technology for sensitive features and equipment failures, applied in nuclear methods, alarms, character and pattern recognition, etc., can solve problems such as low accuracy rate and narrow application range, so as to improve accuracy rate, solve feature failure, and realize early detection and early detection The effect of maintenance
- Summary
- Abstract
- Description
- Claims
- Application Information
AI Technical Summary
Problems solved by technology
Method used
Image
Examples
Embodiment 1
[0076] Example 1, such as figure 1 As shown, a method for early warning of equipment failure based on multi-modal sensitive feature selection and fusion, the specific steps are as follows:
[0077] Step 1: Collect the parameter operation data of the normal state of the equipment under different working conditions, where the parameter operation data includes vibration data, acoustic emission data, temperature data, video monitoring data, electrical signal data and thermal imaging data; during the long-term operation of the equipment In the process, historical sample data is accumulated, including vibration data, acoustic emission data, electrical signal data, video surveillance data, and thermal imaging data. Through data regularization, data cleaning and denoising, removal of outliers and missing values, etc., high-quality structured data is formed, and a multi-modal database that can be retrieved at any time is established. The multimodal database constructed such as figur...
Embodiment 2
[0127] Example 2, such as Figure 5 As shown, a device failure early warning system based on multimodal sensitive feature selection and fusion, including multimodal data acquisition module, industrial Internet of things data transmission module, database module, data format standardization and data statistics information visualization module, multimodal State feature extraction module, feature visualization module and early warning module; the multimodal data acquisition module is connected with the industrial Internet of Things data transmission module, the industrial Internet of Things data transmission module is connected with the database module, and the database module is respectively connected with the data format standardization and The data statistical information visualization module is connected with the multimodal feature extraction module, the multimodal feature extraction module is connected with the feature visualization module, and the feature visualization modul...
PUM
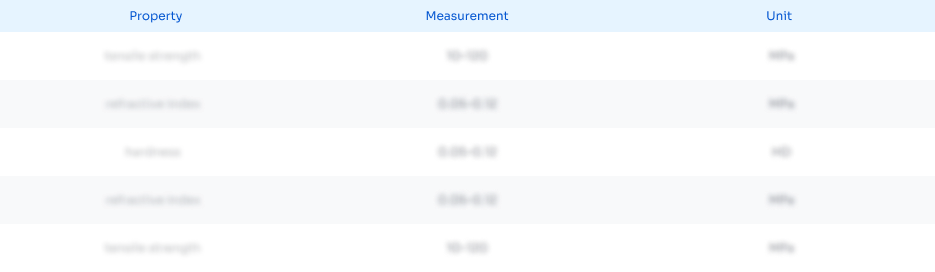
Abstract
Description
Claims
Application Information
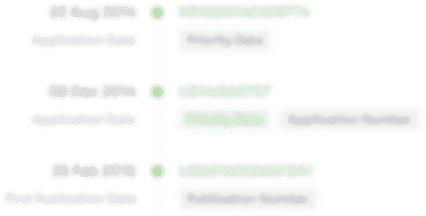
- R&D
- Intellectual Property
- Life Sciences
- Materials
- Tech Scout
- Unparalleled Data Quality
- Higher Quality Content
- 60% Fewer Hallucinations
Browse by: Latest US Patents, China's latest patents, Technical Efficacy Thesaurus, Application Domain, Technology Topic, Popular Technical Reports.
© 2025 PatSnap. All rights reserved.Legal|Privacy policy|Modern Slavery Act Transparency Statement|Sitemap|About US| Contact US: help@patsnap.com