Dangerous article detection method and system based on deep learning model
A technology of deep learning and detection method, which is applied in the field of intelligent detection and intelligent detection of dangerous goods, which can solve the problems that the optical lens cannot capture images, etc., and achieve the effect of broadening the practical range, reducing the interference of decorative textures, and precise frame selection positions
- Summary
- Abstract
- Description
- Claims
- Application Information
AI Technical Summary
Problems solved by technology
Method used
Image
Examples
Embodiment 1
[0036] This embodiment discloses a dangerous goods detection method based on a deep learning model, such as figure 1 shown, including the following steps:
[0037] Under the irradiation of an infrared light source, the S1 simultaneously collects images through an optical lens and an infrared lens, and fuses the optical image and the infrared image to obtain a fused image.
[0038] In this step, it is first necessary to determine the quantity and type of detection targets. According to the material information such as chemical materials, building materials and other materials used on the construction site, determine the specific targets that need to be tested. Count the number of items that need to be detected. Determine the image acquisition device used. According to the distance between the detection target and the detection instrument, determine the clear image of the image captured by the camera. The higher the clarity of the camera, the better the effect of detecting sm...
Embodiment 2
[0072] Based on the same inventive concept, this embodiment discloses a dangerous goods detection system based on a deep learning model, including:
[0073]The fusion module is used to simultaneously collect images through the optical lens and the infrared lens, and fuse the optical image and the infrared image to obtain a fusion image;
[0074] A calibration module is used to calibrate the fused image and give a corresponding label;
[0075] The pre-training module is used to set the initial parameters of the model according to the label, and use the calibrated fusion image to pre-train the model;
[0076] The secondary training module is used to verify the trained model, statistically detect the wrong fusion image, supplement the image according to its characteristics, and use the fusion image and the supplemented image to perform secondary training on the model to obtain the best detection Model; detection module, which is used to fuse the images collected by the optical l...
PUM
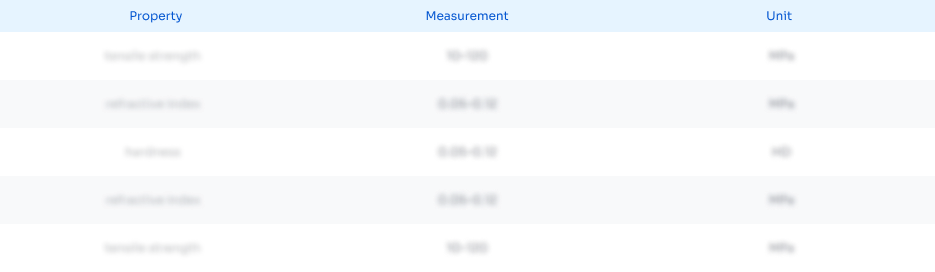
Abstract
Description
Claims
Application Information
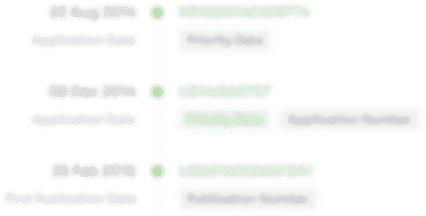
- R&D Engineer
- R&D Manager
- IP Professional
- Industry Leading Data Capabilities
- Powerful AI technology
- Patent DNA Extraction
Browse by: Latest US Patents, China's latest patents, Technical Efficacy Thesaurus, Application Domain, Technology Topic, Popular Technical Reports.
© 2024 PatSnap. All rights reserved.Legal|Privacy policy|Modern Slavery Act Transparency Statement|Sitemap|About US| Contact US: help@patsnap.com