Face super-resolution method based on frequency decomposition multi-attention mechanism
A frequency decomposition and attention technology, applied in the fields of computer vision and image processing, can solve difficult problems
- Summary
- Abstract
- Description
- Claims
- Application Information
AI Technical Summary
Problems solved by technology
Method used
Image
Examples
Embodiment
[0051] Taking 8 times image super-resolution as an example, a face super-resolution method based on frequency decomposition and multi-attention mechanism, for low-resolution face images, more high-frequency components are lost, so for different frequencies The characteristics need to be treated differently. The high-frequency part is processed by complex operations, and the low-frequency part is processed by cheap operations, so that the characteristics of the image can be better restored under the same calculation amount. Specifically, the following steps are included:
[0052] Step S1, such as figure 1 As shown, the input image resolution is a face image of 16×16, and the face image is passed through a 3×3×16×1 convolutional layer, 3×3 represents the size of the convolution kernel, and 16 represents the number of convolution kernels. The last bit represents the movement stride of the convolution kernel, and then the feature map of each channel is decomposed into four downsam...
PUM
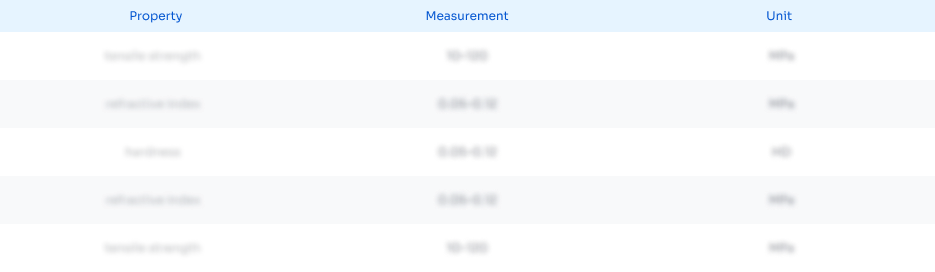
Abstract
Description
Claims
Application Information
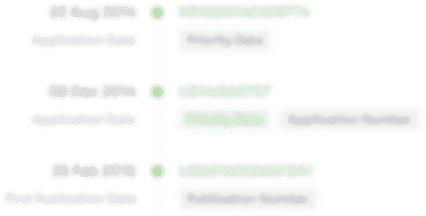
- Generate Ideas
- Intellectual Property
- Life Sciences
- Materials
- Tech Scout
- Unparalleled Data Quality
- Higher Quality Content
- 60% Fewer Hallucinations
Browse by: Latest US Patents, China's latest patents, Technical Efficacy Thesaurus, Application Domain, Technology Topic, Popular Technical Reports.
© 2025 PatSnap. All rights reserved.Legal|Privacy policy|Modern Slavery Act Transparency Statement|Sitemap|About US| Contact US: help@patsnap.com