Air quality prediction model and method based on improved LSTM
A technology for air quality and forecasting models, applied in forecasting, neural learning methods, biological neural network models, etc., can solve problems such as forecast accuracy decline, convergence speed delay, model prediction accuracy decline, etc., to improve forecast accuracy and enhance inclusiveness. , the effect of performance improvement
- Summary
- Abstract
- Description
- Claims
- Application Information
AI Technical Summary
Problems solved by technology
Method used
Image
Examples
Embodiment 1
[0034] This embodiment provides an air quality prediction model based on improved V-LSTM, see figure 1 , the model includes: a multi-channel data input module, a deep learning network module and a multi-path result output module; each module is connected in sequence; wherein, the multi-path result output module selects the corresponding output according to the type of the site to which the corresponding input data belongs Channel input prediction result.
[0035] The present application further improves the use of historical information, and finally, by dislocating the cell state on the output gate, on the one hand, the use frequency of historical information is enhanced, and on the other hand, the training process of the model is stabilized and the accuracy is improved. Then use this depth module as the core to design the overall model such as figure 1 As shown in the figure, multi-channel input and multi-output modules are added to improve the efficiency of multi-channel in...
PUM
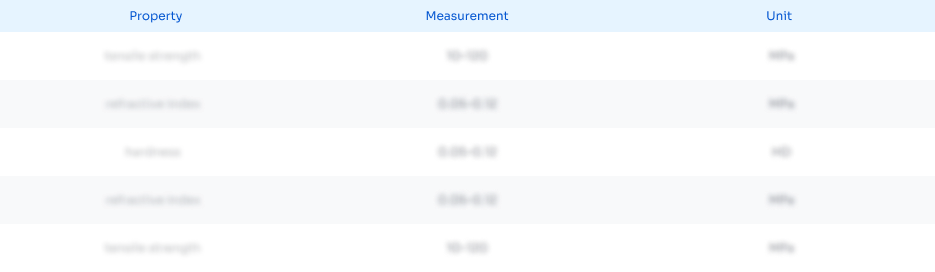
Abstract
Description
Claims
Application Information
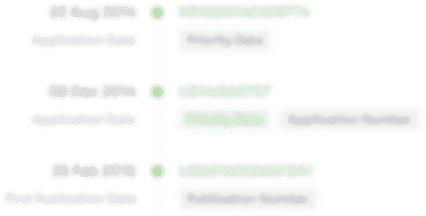
- R&D Engineer
- R&D Manager
- IP Professional
- Industry Leading Data Capabilities
- Powerful AI technology
- Patent DNA Extraction
Browse by: Latest US Patents, China's latest patents, Technical Efficacy Thesaurus, Application Domain, Technology Topic.
© 2024 PatSnap. All rights reserved.Legal|Privacy policy|Modern Slavery Act Transparency Statement|Sitemap