Cross-modal image audio retrieval method based on deep heterogeneous correlation learning
A cross-modal and audio technology, applied in the field of cross-modal image and audio retrieval based on deep heterogeneous correlation learning, can solve a large amount of storage space, insufficient utilization of heterogeneous correlation relations, inability to effectively select cross-modal paired samples, etc. problem, to achieve the effect of improving retrieval accuracy and reducing quantization error
- Summary
- Abstract
- Description
- Claims
- Application Information
AI Technical Summary
Problems solved by technology
Method used
Image
Examples
Embodiment 1
[0048] The environment used in this embodiment is GeForce GTX Titan X GPU, Inter Core i7-5930K, 3.50GHZ CPU, 64G RAM, linux operating system, using Python and open source library KERAS for development.
[0049] The first step is to divide the training data set and test data set:
[0050] Using the Mirflickr 25K image and audio data set, make 50,000 pairs of positive and negative sample image and audio pairs, and select 40,000 pairs as the training data set I train , and the remaining 10,000 pairs are used as the test data set I test ;
[0051] In the second step, pairs of pairs of samples are selected using the cross-modal pairing structure:
[0052] First construct N pairs of binary sample sets and the corresponding set of two-tuple labels Sample set of binary groups Consists of positive sample pairs and negative sample pairs, I i Indicates the i-th picture, V i Indicates the i-th audio, label y i ∈{0,1}, a label of 1 indicates that the image and audio are semantic...
PUM
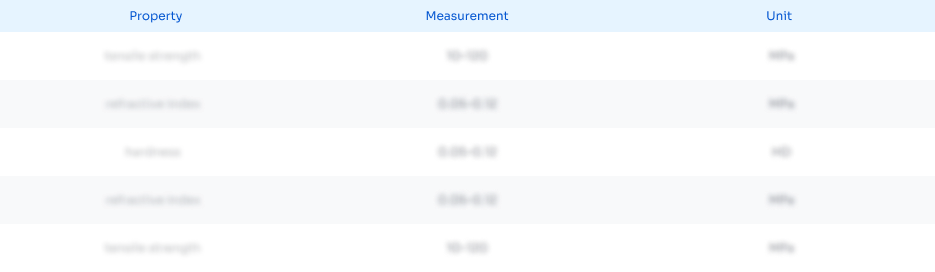
Abstract
Description
Claims
Application Information
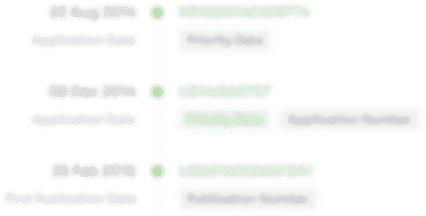
- R&D
- Intellectual Property
- Life Sciences
- Materials
- Tech Scout
- Unparalleled Data Quality
- Higher Quality Content
- 60% Fewer Hallucinations
Browse by: Latest US Patents, China's latest patents, Technical Efficacy Thesaurus, Application Domain, Technology Topic, Popular Technical Reports.
© 2025 PatSnap. All rights reserved.Legal|Privacy policy|Modern Slavery Act Transparency Statement|Sitemap|About US| Contact US: help@patsnap.com