Graph model intelligent commodity recommendation method fusing knowledge graph and user interaction
A knowledge graph and product recommendation technology, applied in the field of data mining recommendation system based on graph convolution, can solve the problems of limited number of products, difficult connection paths, and reduced recommendation accuracy, so as to reduce adverse effects, improve modeling quality, The effect of increasing diversity
- Summary
- Abstract
- Description
- Claims
- Application Information
AI Technical Summary
Problems solved by technology
Method used
Image
Examples
Embodiment Construction
[0036]In this embodiment, a graphical model intelligent product recommendation method that integrates knowledge graphs and user interactions is to map users, products, and nodes and associations in knowledge graphs into a latent semantic vector space with the same dimension, and then use graph The method of feature propagation and weighted combination in the convolutional network aggregates the feature vectors that affect each other, so that each node can transfer knowledge information to each other, automatically integrate the rich semantic information and related information in the knowledge graph, and condense it into user expression and product expression. , make full use of the additional information contained in the user's historical interaction records and knowledge graphs, so as to achieve more accurate personalized product recommendations. Specifically, if figure 1 As shown, proceed as follows:
[0037] Step 1. Collect and preprocess the user’s historical interaction...
PUM
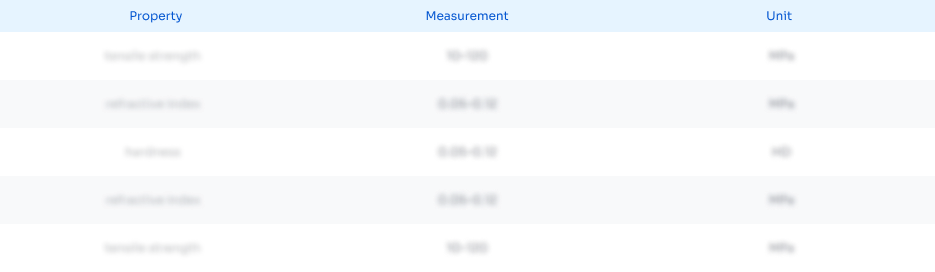
Abstract
Description
Claims
Application Information
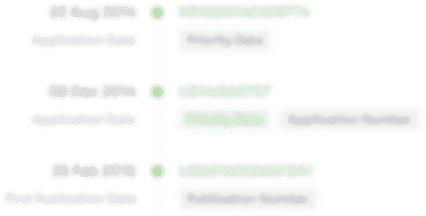
- R&D Engineer
- R&D Manager
- IP Professional
- Industry Leading Data Capabilities
- Powerful AI technology
- Patent DNA Extraction
Browse by: Latest US Patents, China's latest patents, Technical Efficacy Thesaurus, Application Domain, Technology Topic, Popular Technical Reports.
© 2024 PatSnap. All rights reserved.Legal|Privacy policy|Modern Slavery Act Transparency Statement|Sitemap|About US| Contact US: help@patsnap.com