Federal learning method and device for credit card fraud prevention
A learning method and credit card technology, applied in the field of credit card anti-fraud federated learning methods and devices, can solve the problems of inaccurate learning, difficulty in obtaining high-performance models from data, and failure to consider local model personalization, etc., to improve training accuracy , improve accuracy, and improve the effect of anti-fraud assessment accuracy
- Summary
- Abstract
- Description
- Claims
- Application Information
AI Technical Summary
Problems solved by technology
Method used
Image
Examples
Embodiment 1
[0061] Figure 5 This is a flowchart of a federated learning method for credit card anti-fraud according to an embodiment of the present invention. This embodiment can be applied to the situation of identifying the credit card fraud category through equipment such as a server, and the method can be executed by a federated learning device for credit card anti-fraud, which can be implemented in software and / or hardware, and can be integrated in In electronic equipment, such as integrated server equipment.
[0062] The federated learning method is mainly used to identify the two most common types of credit card fraud. Type 1 is stolen card fraud: fraudulent use or misappropriation of lost credit cards for transactions; type 2 is virtual application fraud: applicants use false information to apply for credit cards , to avoid the card issuer's review. Therefore, the goal of the federated learning method proposed in this embodiment is to improve the accuracy of the three-classific...
Embodiment 2
[0106] The embodiment of the present invention proposes a federated learning device for credit card anti-fraud. The federated learning device includes a local model building module, a federated learning training module and a global graph convolutional neural network model.
[0107] The local model building module is used to build the local graph convolutional neural network model corresponding to K federated learning participants with different fraud categories; the local undirected graph structure data owned by each participant is G i (V,E,A)(i∈K), where the set of nodes in the graph structure is v i ∈V, v i The feature on the node is x i ∈X, each node contains a variety of key feature information including user information, loan amount, deposit amount and credit data, the edge set between nodes is e i,j =(v i ,v j ) ∈ E; A represents the adjacency matrix, which defines the mutual connection between nodes; the fraud categories include stolen card fraud, virtual applicatio...
PUM
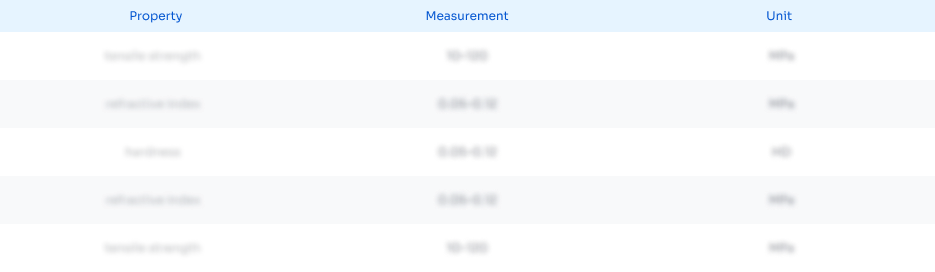
Abstract
Description
Claims
Application Information
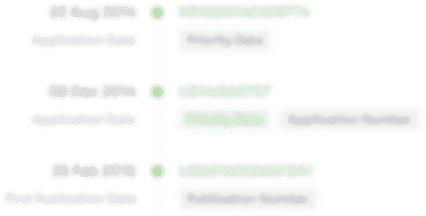
- R&D
- Intellectual Property
- Life Sciences
- Materials
- Tech Scout
- Unparalleled Data Quality
- Higher Quality Content
- 60% Fewer Hallucinations
Browse by: Latest US Patents, China's latest patents, Technical Efficacy Thesaurus, Application Domain, Technology Topic, Popular Technical Reports.
© 2025 PatSnap. All rights reserved.Legal|Privacy policy|Modern Slavery Act Transparency Statement|Sitemap|About US| Contact US: help@patsnap.com