A sequential social recommendation method, system and storage medium based on gate mechanism
A recommendation method and sequence technology, applied in the field of recommendation systems, can solve the problems of inaccurate interest learning and insufficient expression of users' real interests.
- Summary
- Abstract
- Description
- Claims
- Application Information
AI Technical Summary
Problems solved by technology
Method used
Image
Examples
Embodiment 1
[0054] A sequential social recommendation method based on gate mechanism, the social recommendation method includes:
[0055] Step S1, divide the user's original consumption data and the friend's original consumption data into sequences respectively, obtain the user's sequence segment and the friend's sequence segment, initialize the user's sequence segment and the friend's sequence segment, and obtain the user's sequence data for identification by the GRU neural network and friend sequence data;
[0056] Step S2, based on the GRU neural network of the selection gate mechanism, filter and select the user sequence data to obtain the current interests of the user, and filter and select the friend sequence data to obtain the current interests of the friends;
[0057] Step S3, splicing the current interests of the friends to obtain the short-term interests of the friends, initializing the commodity data in the original consumption data of the friends, obtaining the long-term inter...
Embodiment 2
[0109] For ease of understanding, this embodiment uses a specific example to describe the sequential social recommendation method based on the gate mechanism, such as image 3 As shown, the sequential social recommendation method based on the gate mechanism includes:
[0110] S1, the user sequence data x u =(m 1 ,m 2 ,...m j ) is input to the GRU neural network, and multiple hidden states h are obtained in sequence corresponding to the sequence data. n , multiple hidden states h n Filter the selection through the selection gate to obtain the user's current interest Output obtain the user's current interest
[0111] S2, the friend sequence data x f =(k 1 ,k 2 ,...k j) is input to the GRU neural network, corresponding to the sequence data sequence to obtain multiple hidden states h f , the friend sequence data and the h f The last hidden state h of g Filter selection to get current interests of friends put h g current interests with friends Splicing to get int...
Embodiment 3
[0117] This embodiment provides a sequential social recommendation system based on a gate mechanism, such as Figure 4 As shown, including: initial module, interest acquisition module and training module;
[0118] The initial module is used to divide the user's original consumption data and the friend's original consumption data into sequences respectively, obtain the user sequence segment and the friend sequence segment, initialize the user sequence segment and the friend sequence segment, and obtain the sequence for the GRU neural network to identify. User sequence data and friend sequence data of ;
[0119] The interest acquisition module is used to filter and select the user sequence data based on the GRU neural network of the selection gate mechanism to obtain the current interests of the user, and filter and select the friend sequence data to obtain the current interests of the friends; Perform splicing processing on the current interests of the friends, obtain the shor...
PUM
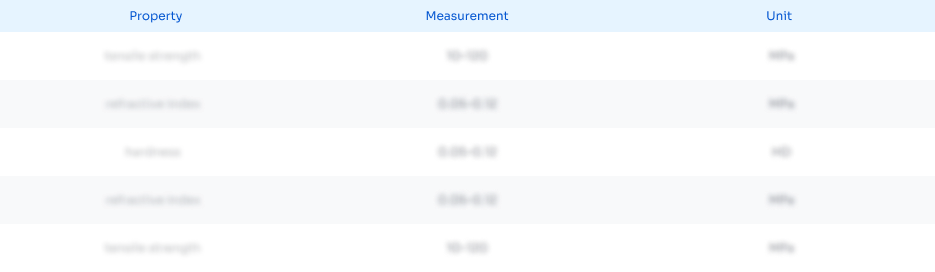
Abstract
Description
Claims
Application Information
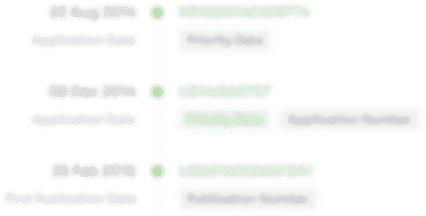
- R&D
- Intellectual Property
- Life Sciences
- Materials
- Tech Scout
- Unparalleled Data Quality
- Higher Quality Content
- 60% Fewer Hallucinations
Browse by: Latest US Patents, China's latest patents, Technical Efficacy Thesaurus, Application Domain, Technology Topic, Popular Technical Reports.
© 2025 PatSnap. All rights reserved.Legal|Privacy policy|Modern Slavery Act Transparency Statement|Sitemap|About US| Contact US: help@patsnap.com