Harmonic reducer fault diagnosis method and system based on generative adversarial network
A harmonic reducer, fault diagnosis technology, applied in the direction of biological neural network models, instruments, mechanical parts testing, etc., can solve the problem that the harmonic reducer is not applicable, the diagnosis effect needs to be improved, and it is difficult to reflect the operation status of the harmonic reducer and other issues to achieve the effect of improving accuracy
- Summary
- Abstract
- Description
- Claims
- Application Information
AI Technical Summary
Problems solved by technology
Method used
Image
Examples
Embodiment
[0029] Such as figure 1 As shown, the fault diagnosis method of harmonic reducer based on generative confrontation network in this embodiment mainly includes the following steps:
[0030] S1. Perform data preprocessing, collect the vibration acceleration signals of the harmonic reducer through three vibration acceleration sensors, use the fast Fourier transform FFT to process the data to extract the characteristics of the original signal, and use the normalized data to construct the original data set to for subsequent data generation;
[0031] S2. Perform data generation, use the generative confrontation network GAN composed of the convolutional layer and the fully connected layer to enhance various scarce fault data, and generate multiple types of fault data by using multiple generative confrontation network GANs;
[0032] S3. Perform data selection, use the data selection module composed of data filtering and data purification to filter and purify the generated data, filter...
PUM
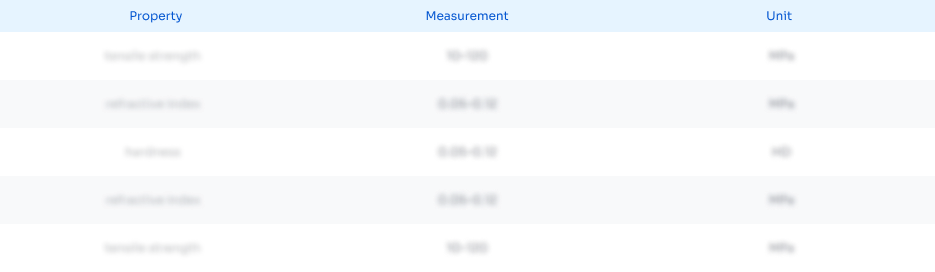
Abstract
Description
Claims
Application Information
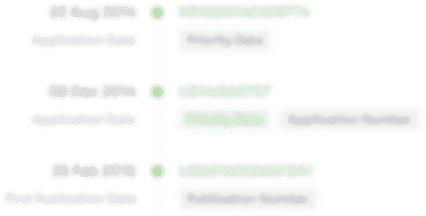
- R&D
- Intellectual Property
- Life Sciences
- Materials
- Tech Scout
- Unparalleled Data Quality
- Higher Quality Content
- 60% Fewer Hallucinations
Browse by: Latest US Patents, China's latest patents, Technical Efficacy Thesaurus, Application Domain, Technology Topic, Popular Technical Reports.
© 2025 PatSnap. All rights reserved.Legal|Privacy policy|Modern Slavery Act Transparency Statement|Sitemap|About US| Contact US: help@patsnap.com