Subway passenger flow multi-step prediction method based on space-time parallel grid neural network
A neural network and multi-step forecasting technology, applied in neural learning methods, biological neural network models, forecasting, etc., can solve the spatial characteristics of dynamic characteristics that are difficult to static, cannot express the spatial correlation of subway stations, and cannot properly describe the transfer of subway stations Flow and other issues, to achieve the effect of convenient use and reasonable structure
- Summary
- Abstract
- Description
- Claims
- Application Information
AI Technical Summary
Problems solved by technology
Method used
Image
Examples
Embodiment Construction
[0064] The present invention will be described in detail below in conjunction with specific embodiments. The following examples will help those skilled in the art to further understand the present invention, but do not limit the present invention in any form. It should be noted that those skilled in the art can make several changes and improvements without departing from the concept of the present invention. These all belong to the protection scope of the present invention.
[0065] Such as figure 1 As shown, the present invention is a multi-step prediction method of subway passenger flow based on spatio-temporal parallel grid neural network. Specifically, this embodiment includes the following steps:
[0066] Step A: Learn the time relationship of subway traffic flow through the grid neural network, and capture the short-term temporal correlation of subway traffic flow based on the short-term historical subway traffic flow and the neighbor grid neural network; including th...
PUM
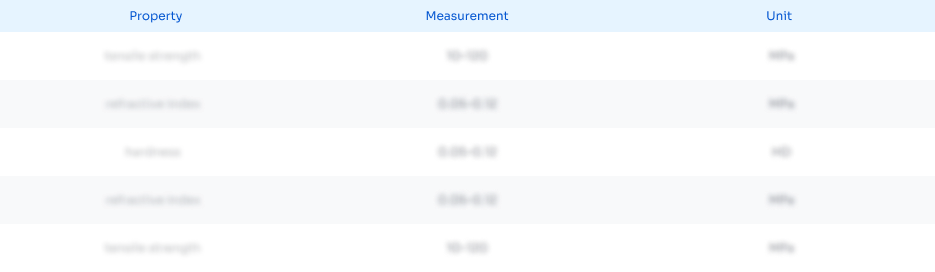
Abstract
Description
Claims
Application Information
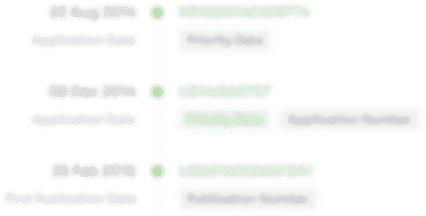
- R&D
- Intellectual Property
- Life Sciences
- Materials
- Tech Scout
- Unparalleled Data Quality
- Higher Quality Content
- 60% Fewer Hallucinations
Browse by: Latest US Patents, China's latest patents, Technical Efficacy Thesaurus, Application Domain, Technology Topic, Popular Technical Reports.
© 2025 PatSnap. All rights reserved.Legal|Privacy policy|Modern Slavery Act Transparency Statement|Sitemap|About US| Contact US: help@patsnap.com