Multi-view comparative learning-based citation network graph representation learning system and method
A technology of learning system and network diagram, applied in the field of citation network diagram representation learning system based on multi-view contrast learning, can solve the problems of ignoring position information, confusion, limited recognition ability of diagram representation, etc. Effect
- Summary
- Abstract
- Description
- Claims
- Application Information
AI Technical Summary
Problems solved by technology
Method used
Image
Examples
Embodiment 1
[0075] This embodiment provides a citation network graph representation learning system based on multi-view comparative learning, including:
[0076] The sample construction module 11 is used to represent the corresponding original graph node in the citation network graph as a positive sample, and construct a negative corresponding sample based on the original graph;
[0077] The graph enhancement module 12 is used to enhance the graph structure and initial node features in the positive sample based on the personalized page ranking algorithm and the Laplac smoothing algorithm, and obtain positive sample graphs and negative sample graphs related to the positive sample node set;
[0078] The fusion module 13 is used to extract positive sample graph representations and negative sample graph representations based on the GCN encoder, integrate positive sample graph representations and negative sample graph representations, and centralize the fusion layer through cross-views to obtai...
Embodiment 2
[0147] The difference between the citation network graph representation learning system based on multi-view comparative learning provided in this embodiment and the first embodiment is that:
[0148] To evaluate the effectiveness of the proposed MHGI, extensive experiments are conducted on six widely used datasets, including Cora1, Citeseer1, Pubmed1, Amap2, Amac2 and Corafull3. For the Cora, CiteSeer and PubMed datasets, the same train / validation / test split was used as in [Thomas N.Kipf and MaxWelling.2017.Semi-Supervised Classification with Graph ConvolutionalNetworks.InProceedings of the International Conference on Learning Representations]. For the other three datasets (i.e. Amap, Amac, and Corafull), since they have no common split criteria available, random splits are used, where 7%, 7%, and the remaining 86% of nodes are selected for training, validation, and testing, respectively set.
[0149] Table 1 shows the comparison of node classification accuracy of different m...
PUM
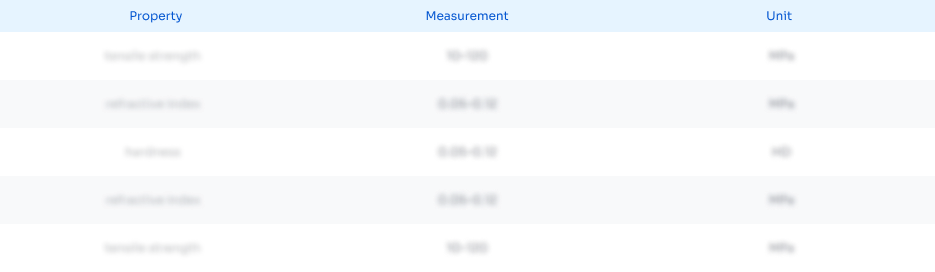
Abstract
Description
Claims
Application Information
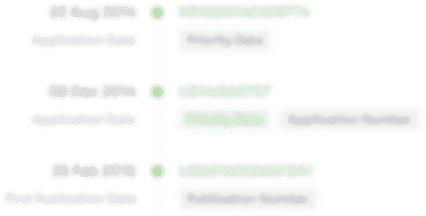
- Generate Ideas
- Intellectual Property
- Life Sciences
- Materials
- Tech Scout
- Unparalleled Data Quality
- Higher Quality Content
- 60% Fewer Hallucinations
Browse by: Latest US Patents, China's latest patents, Technical Efficacy Thesaurus, Application Domain, Technology Topic, Popular Technical Reports.
© 2025 PatSnap. All rights reserved.Legal|Privacy policy|Modern Slavery Act Transparency Statement|Sitemap|About US| Contact US: help@patsnap.com