Coastline change analysis method based on deep learning
A technology of change analysis and deep learning, applied in the field of coastline change analysis, can solve problems such as different shoreline extraction criteria, complicated shoreline types, and difficulty in achieving accurate classification of shoreline types, and achieve enhanced effects and improved extraction accuracy.
- Summary
- Abstract
- Description
- Claims
- Application Information
AI Technical Summary
Problems solved by technology
Method used
Image
Examples
specific Embodiment approach 1
[0066] Specific implementation mode one: combine figure 1 Describe this embodiment, the specific process of a coastline change analysis method based on deep learning in this embodiment is:
[0067] Step 1. Select the coastline data set (multi-temporal coastline remote sensing image and corresponding coastline type (such as artificial coastline type, etc.)) of the coastline data set in the research area, and divide the coastline data set into a training set and a test set;
[0068] Construct a neural network, input the training set into the neural network for training, and obtain the trained network, input the test set into the neural network for testing, and output the coastline type. If the test results meet the requirements, a trained neural network is obtained. If the test results do not meet the requirements Then retrain by adjusting model parameters or training data sets until a trained neural network is obtained;
[0069] The training set includes multi-temporal coastli...
specific Embodiment approach 2
[0075] Specific embodiment two: the difference between this embodiment and specific embodiment one is: the coastline data set of the research area is selected in the step one, and the coastline data set is divided into a training set and a test set;
[0076] Construct a neural network, input the training set into the neural network for training, and obtain the trained network, input the test set into the neural network for testing, and output the coastline type. If the test results meet the requirements, a trained neural network is obtained. If the test results do not meet the requirements Then retrain by adjusting model parameters or training data sets until a trained neural network is obtained;
[0077] The specific process is:
[0078] Step 11, select the coastline data set of the research area, and divide the coastline data set into a training set and a test set, wherein the ratio of the training set to the test set is 4:1;
[0079] Step 1 and 2, construct VGG16, MobileNe...
specific Embodiment approach 3
[0083] Embodiment 3: This embodiment differs from Embodiment 1 or Embodiment 2 in that: in the step 2, the multi-temporal coastline remote sensing image is processed to obtain the processed multi-temporal coastline remote sensing image;
[0084] Input the processed multi-temporal coastline remote sensing image into the trained neural network to obtain the coastline type corresponding to the processed multi-temporal coastline remote sensing image; the specific process is as follows:
[0085] Perform image deaverage and clipping on the multi-temporal coastline remote sensing image to obtain the remote sensing image area of interest;
[0086] Input the obtained remote sensing image area of interest into a suitable trained neural network according to actual needs (for example, the MobileNet network can provide the fastest training speed, the Inception-ResNet network can provide the highest test set accuracy, etc.), and the processed The coastline types of multi-temporal coastl...
PUM
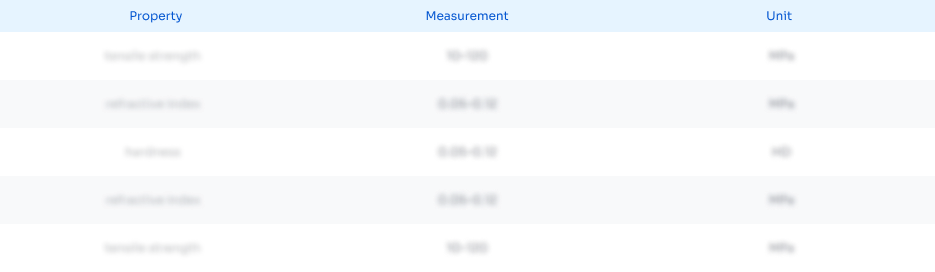
Abstract
Description
Claims
Application Information
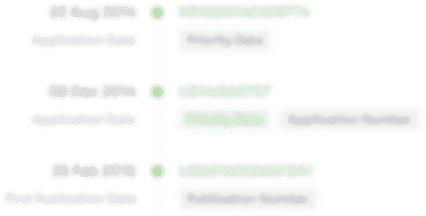
- R&D Engineer
- R&D Manager
- IP Professional
- Industry Leading Data Capabilities
- Powerful AI technology
- Patent DNA Extraction
Browse by: Latest US Patents, China's latest patents, Technical Efficacy Thesaurus, Application Domain, Technology Topic, Popular Technical Reports.
© 2024 PatSnap. All rights reserved.Legal|Privacy policy|Modern Slavery Act Transparency Statement|Sitemap|About US| Contact US: help@patsnap.com