Zero sample image classification method based on generative adversarial network
A technology of sample images and classification methods, applied in the field of deep learning, can solve the problems of single image features, collapse of adversarial network mode, low classification accuracy, etc., to achieve diversification of visual image features, improve classification accuracy, and close correlation. Effect
- Summary
- Abstract
- Description
- Claims
- Application Information
AI Technical Summary
Problems solved by technology
Method used
Image
Examples
Embodiment Construction
[0045] In order to make the object, technical solution and advantages of the present invention clearer, the present invention will be described in detail below in conjunction with the accompanying drawings and specific embodiments.
[0046] The present invention provides a zero-sample image classification method based on a generative adversarial network. By generating visual image features of unknown classes, the zero-sample classification task is transformed into a traditional image classification task. At the same time, the generator network in the generative adversarial network is Improvements are made to make the generated visual image features more realistic, thereby further improving the quality of the generated visual image features; and then the image features are used to locate the key information in the visual image features through the attention network, ignoring other interference information, so as to train The classifier enables the generator to generate visual im...
PUM
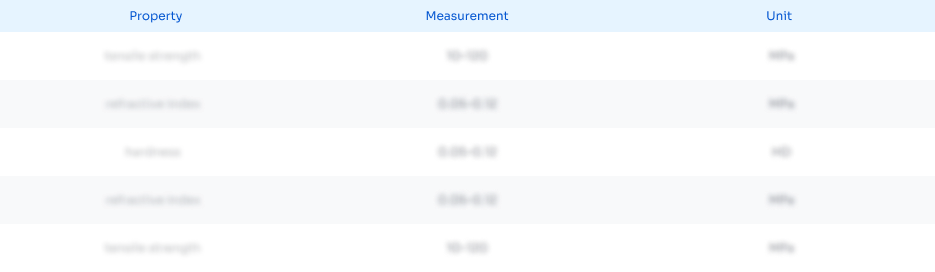
Abstract
Description
Claims
Application Information
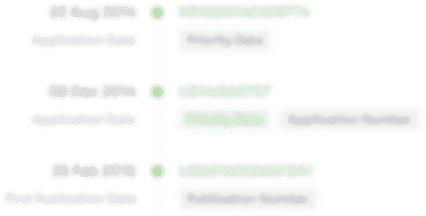
- R&D
- Intellectual Property
- Life Sciences
- Materials
- Tech Scout
- Unparalleled Data Quality
- Higher Quality Content
- 60% Fewer Hallucinations
Browse by: Latest US Patents, China's latest patents, Technical Efficacy Thesaurus, Application Domain, Technology Topic, Popular Technical Reports.
© 2025 PatSnap. All rights reserved.Legal|Privacy policy|Modern Slavery Act Transparency Statement|Sitemap|About US| Contact US: help@patsnap.com