topological perception post-processing confidence coefficient correction method applied to GNN
A correction method and confidence level technology, applied in neural learning methods, biological neural network models, design optimization/simulation, etc., can solve problems such as overconfidence in prediction, low prediction accuracy of the model, and inability to be added to the training set, etc., to improve The effect of confidence
- Summary
- Abstract
- Description
- Claims
- Application Information
AI Technical Summary
Problems solved by technology
Method used
Image
Examples
Embodiment Construction
[0037] In this invention, we focus on correcting the undirected property graph G=(V,E) with the adjacency matrix A∈R N*N And node feature matrix X=[x 1 ,...,x N ]. V is a node set, E∈V*V is an edge set composed of edges between nodes, and N=|V| is the number of nodes. We give the definition of GNN being perfectly corrected:
[0038] Definition 1: Given a random variable and a GNN model f θ , where θ is a learnable parameter, and for node i, define its label as y i ∈ Y, the output of the GNN is z i = f θ (x i , A)=(z i,1 ,...,z i,K ), and define with are the prediction of the model and the corresponding confidence, then we say that when f θ It is perfectly corrected when it satisfies the following definition:
[0039]
[0040] According to Definition 1, only when the confidence GNNs are perfectly calibrated when exactly equal to the true probability of each node getting a correct prediction.
[0041] Next, we take two representative GNNs (GCN and GAT) as ...
PUM
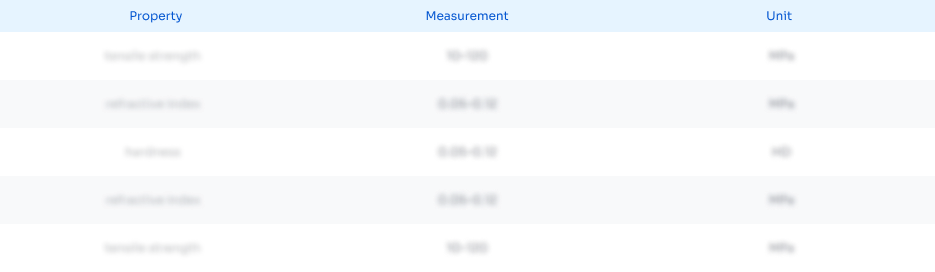
Abstract
Description
Claims
Application Information
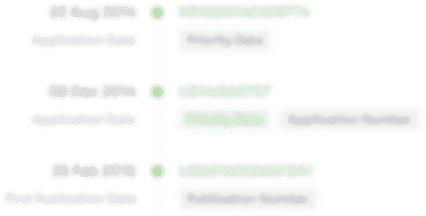
- R&D
- Intellectual Property
- Life Sciences
- Materials
- Tech Scout
- Unparalleled Data Quality
- Higher Quality Content
- 60% Fewer Hallucinations
Browse by: Latest US Patents, China's latest patents, Technical Efficacy Thesaurus, Application Domain, Technology Topic, Popular Technical Reports.
© 2025 PatSnap. All rights reserved.Legal|Privacy policy|Modern Slavery Act Transparency Statement|Sitemap|About US| Contact US: help@patsnap.com