Multi-modal chemical process fault detection method based on improved t-SNE
A chemical process and fault detection technology, which is applied in the direction of program control, comprehensive factory control, comprehensive factory control, etc., can solve the problems affecting the control limit estimation and fault detection effect, and achieve the effect of high accuracy and strong robustness
- Summary
- Abstract
- Description
- Claims
- Application Information
AI Technical Summary
Problems solved by technology
Method used
Image
Examples
Embodiment Construction
[0093] The following will clearly and completely describe the technical solutions in the embodiments of the present invention with reference to the drawings in the embodiments of the present invention. Obviously, the described embodiments are part of the embodiments of the present invention, not all of them. Based on the embodiments of the present invention, all other embodiments obtained by persons of ordinary skill in the art without making creative efforts shall fall within the protection scope of the present invention.
[0094] like figure 1 As shown, the present invention provides a multi-modal chemical process fault detection method based on improved t-SNE. First, collect the data of the normal operation of the chemical process of multiple modes, and perform standardized preprocessing to obtain the training data; secondly, calculate the Mahalanobis distance of the training data, and realize multi-modal chemical process modeling by improving the t-SNE algorithm ;Finally,...
PUM
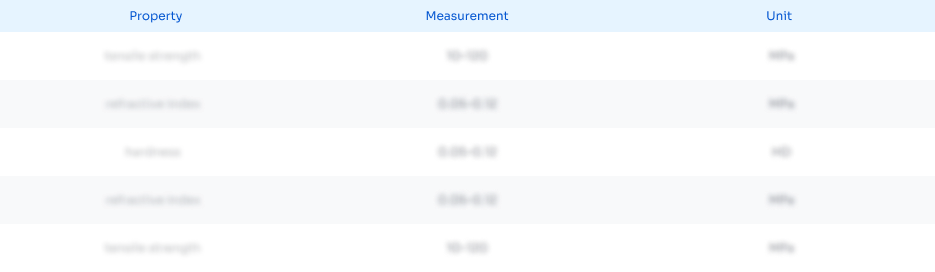
Abstract
Description
Claims
Application Information
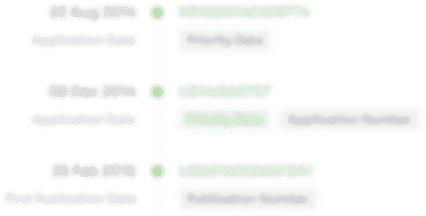
- R&D Engineer
- R&D Manager
- IP Professional
- Industry Leading Data Capabilities
- Powerful AI technology
- Patent DNA Extraction
Browse by: Latest US Patents, China's latest patents, Technical Efficacy Thesaurus, Application Domain, Technology Topic, Popular Technical Reports.
© 2024 PatSnap. All rights reserved.Legal|Privacy policy|Modern Slavery Act Transparency Statement|Sitemap|About US| Contact US: help@patsnap.com