A kurtosis-based fastica and approximation solution domain for stlbo motor bearing fault diagnosis
A technology for motor bearing and fault diagnosis, applied in neural learning methods, testing of computer parts, mechanical parts, etc., can solve problems such as low reliability and practicability, low generalization ability, slow algorithm convergence, etc. High workload, reliability and generalizability, and the effect of improving convergence speed
- Summary
- Abstract
- Description
- Claims
- Application Information
AI Technical Summary
Problems solved by technology
Method used
Image
Examples
Embodiment Construction
[0044] The technical solution of the invention is described in detail below in conjunction with the accompanying drawings:
[0045] like figure 1 The fault method of STLBO motor bearing vibration signal based on kurtosis FastICA and approximation solution domain includes the following steps:
[0046] Step 1, use the accelerometer sensor to collect the vibration acceleration signal of the motor bearing under no fault and different fault conditions, perform whitening and decorrelation preprocessing on the collected one-dimensional vibration signal, and use the kurtosis-based FastICA to whiten Separation from the decorrelated vibration data;
[0047] Step 2, extracting the features of Mel cepstral coefficients on the separated vibration signals, correspondingly labeling the vibration signals of the motor bearing under different working conditions, and then dividing the extracted samples into a training set and a test set;
[0048] Step 3, according to the samples after feature ex...
PUM
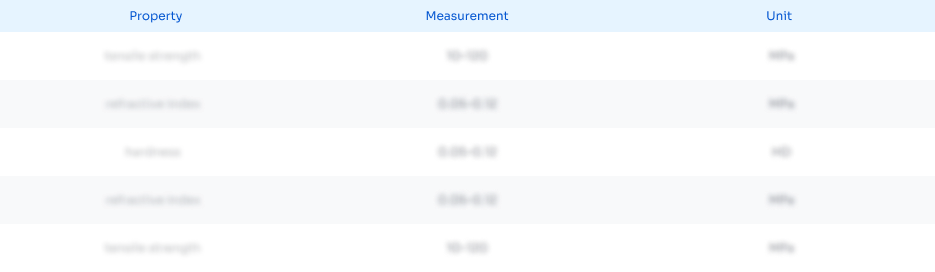
Abstract
Description
Claims
Application Information
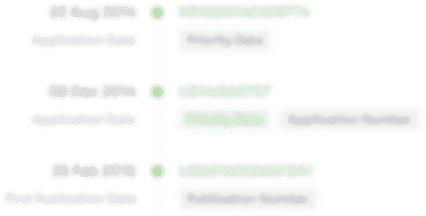
- R&D Engineer
- R&D Manager
- IP Professional
- Industry Leading Data Capabilities
- Powerful AI technology
- Patent DNA Extraction
Browse by: Latest US Patents, China's latest patents, Technical Efficacy Thesaurus, Application Domain, Technology Topic, Popular Technical Reports.
© 2024 PatSnap. All rights reserved.Legal|Privacy policy|Modern Slavery Act Transparency Statement|Sitemap|About US| Contact US: help@patsnap.com