Turbofan engine remaining service life prediction method based on improved stacked sparse auto-encoder and attention echo state network
- Summary
- Abstract
- Description
- Claims
- Application Information
AI Technical Summary
Problems solved by technology
Method used
Image
Examples
Embodiment Construction
[0044] refer to figure 1 , a turbofan engine remaining service life prediction method based on an improved stacked sparse autoencoder and attentional echo state network, including the following steps:
[0045] 1) Data selection is performed on the acquired sensor data generated by different engines over time to form an original data set Each data sample contains the number of the engine in the i-th environment, the running time from the beginning to the present, the operating settings, and the information of the sensor, where i represents the i-th environment, and then the 3sigma of the data is performed on the original data set Noise reduction and normalization processing, 3sigma criterion to eliminate gross errors in the measurement data, that is, the data distribution is almost concentrated in the (μ-3σ, μ+3σ) interval, the proportion is 99.73%, and the proportion of data exceeding this interval is 0.27 %, this part of the data belongs to the coarse error and is considere...
PUM
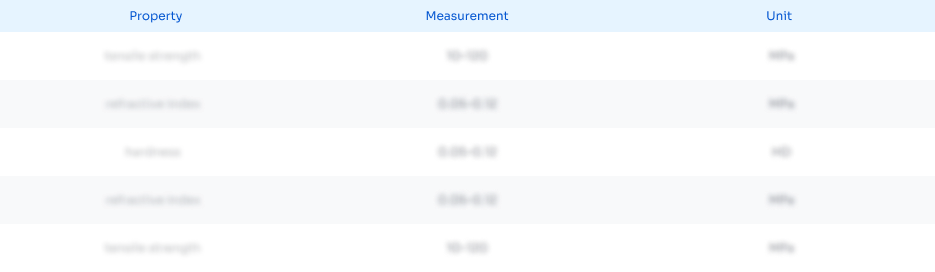
Abstract
Description
Claims
Application Information
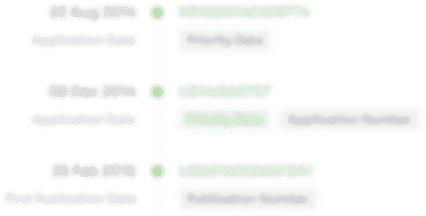
- R&D
- Intellectual Property
- Life Sciences
- Materials
- Tech Scout
- Unparalleled Data Quality
- Higher Quality Content
- 60% Fewer Hallucinations
Browse by: Latest US Patents, China's latest patents, Technical Efficacy Thesaurus, Application Domain, Technology Topic, Popular Technical Reports.
© 2025 PatSnap. All rights reserved.Legal|Privacy policy|Modern Slavery Act Transparency Statement|Sitemap|About US| Contact US: help@patsnap.com