Fine-grained cross-media retrieval method based on unified double-branch network
A branch network and cross-media technology, applied in the field of fine-grained cross-media retrieval based on a unified dual branch network, to achieve the effects of accurate semantic feature representation, low computational cost, and reduced heterogeneity differences
- Summary
- Abstract
- Description
- Claims
- Application Information
AI Technical Summary
Problems solved by technology
Method used
Image
Examples
Embodiment 1
[0043] This embodiment proposes a fine-grained cross-media retrieval method based on a unified dual-branch network, such as figure 1 shown, including the following steps:
[0044] Step 1: use the sample training set to train a unified double-branch network model; the unified double-branch network model includes a unified convolutional neural network feature extraction module, a unified probability feature branch, a media independent feature branch and a cross-media public feature combination module; The unified convolutional neural network features are respectively connected with the unified probability feature branch and the media independent feature branch to extract a unified common convolution feature ; The output ends of the unified probability feature branch and the media independent feature branch are respectively connected with the media independent feature branch; learning; the media-independent feature branch is used to learn the corresponding media types respecti...
Embodiment 2
[0049] In this embodiment, on the basis of the above-mentioned embodiment 1, in order to better realize the present invention, further, the specific operation of the step 3 is as follows:
[0050] Step 3.1: Extract the common convolutional features of the input samples through the unified convolutional neural network feature extraction module ;
[0051] Step 3.2: Combine the obtained common convolutional features Input to the unified probability feature branch and the media independent feature branch respectively;
[0052] Step 3.3: Co-convolve features via the unified probabilistic feature branch process to obtain the uniform probability feature of the input sample ; common convolutional features via media-independent feature branches process to obtain the media-independent features of the input samples ;
[0053] Step 3.4: Set probability correction features ; The probability correction feature Unified probabilistic features for and across media A vector of...
Embodiment 3
[0059] In this embodiment, on the basis of any one of the above-mentioned embodiments 1-2, in order to better realize the present invention, further, the specific operation of the step 3.6 is as follows:
[0060] Step 3.6.1: Use each of the K input samples to correct the features for the probability Update, the specific update operation is: through the same media similarity measurement, obtain the category label of the training sample that is most similar to the input in the database, and modify the probability correction feature The probability value corresponding to the category of a certain real label pair, and the category probability value of a certain real label pair The specific update formula is as follows:
[0061] ;
[0062] Step 3.6.2: Correct the feature with the updated correction probability with uniform probability features weighted combination to get the final cross-media public features , the specific weighted combination formula is as follows:
...
PUM
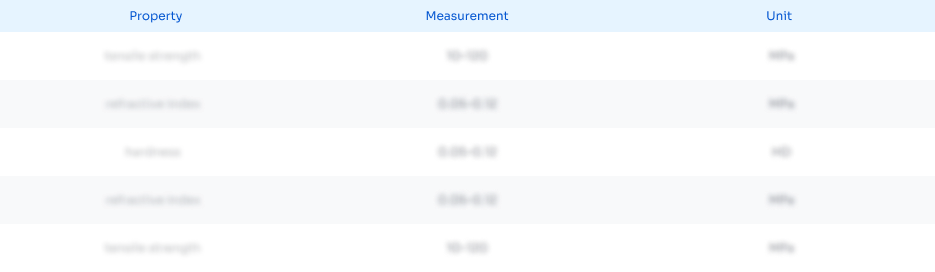
Abstract
Description
Claims
Application Information
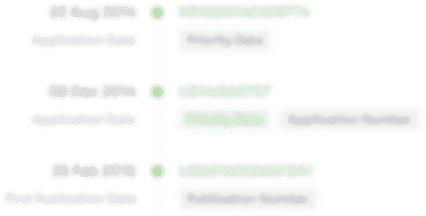
- Generate Ideas
- Intellectual Property
- Life Sciences
- Materials
- Tech Scout
- Unparalleled Data Quality
- Higher Quality Content
- 60% Fewer Hallucinations
Browse by: Latest US Patents, China's latest patents, Technical Efficacy Thesaurus, Application Domain, Technology Topic, Popular Technical Reports.
© 2025 PatSnap. All rights reserved.Legal|Privacy policy|Modern Slavery Act Transparency Statement|Sitemap|About US| Contact US: help@patsnap.com